
Big Data Analytics and Machine Intelligence in Biomedical and Health Informatics
Concepts, Methodologies, Tools and Applications
Sunil Kumar Dhal, Subhendu Kumar Pani, Srinivas Prasad, Sudhir Kumar Mohapatra, Sunil Kumar Dhal, Srinivas Prasad, Sudhir Kumar Mohapatra, Subhendu Kumar Pani
- English
- ePUB (mobile friendly)
- Available on iOS & Android
Big Data Analytics and Machine Intelligence in Biomedical and Health Informatics
Concepts, Methodologies, Tools and Applications
Sunil Kumar Dhal, Subhendu Kumar Pani, Srinivas Prasad, Sudhir Kumar Mohapatra, Sunil Kumar Dhal, Srinivas Prasad, Sudhir Kumar Mohapatra, Subhendu Kumar Pani
About This Book
BIG DATA ANALYTICS AND MACHINE INTELLIGENCE IN BIOMEDICAL AND HEALTH INFORMATICS
Provides coverage of developments and state-of-the-art methods in the broad and diversified data analytics field and applicable areas such as big data analytics, data mining, and machine intelligence in biomedical and health informatics.
The novel applications of Big Data Analytics and machine intelligence in the biomedical and healthcare sector is an emerging field comprising computer science, medicine, biology, natural environmental engineering, and pattern recognition. Biomedical and health informatics is a new era that brings tremendous opportunities and challenges due to the plentifully available biomedical data and the aim is to ensure high-quality and efficient healthcare by analyzing the data.
The 12 chapters in?? Big Data Analytics and Machine Intelligence in Biomedical and Health Informatics??cover the latest advances and developments in health informatics, data mining, machine learning, and artificial intelligence. They have been organized with respect to the similarity of topics addressed, ranging from issues pertaining to the Internet of Things (IoT) for biomedical engineering and health informatics, computational intelligence for medical data processing, and Internet of Medical Things??(IoMT).
New researchers and practitioners working in the field will benefit from reading the book as they can quickly ascertain the best performing methods and compare the different approaches.
Audience
Researchers and practitioners working in the fields of biomedicine, health informatics, big data analytics, Internet of Things, and machine learning.
Frequently asked questions
Information
1
An Introduction to Big Data Analytics Techniques in Healthcare
AbstractThere is a notable rise in the amount of data being generated in the healthcare industries. Trying to improve the health outcomes and cut the costs derived from better utilization of healthcare data has been of great interest to healthcare providers (and the abundance of the data has brought that about big change), whereas the nature of healthcare data presents specific problems when it comes to processing and looking at big data, particularly, as well as analyzing the abundance of it. Some new ideas about how to deal with these problems are discussed in this chapter. According to this chapter, there are two ways in which advances in processing healthcare data have been made in the last 10 years that may make generating better predictions from the medical data feasible. Firstly by using advancing technological methods of analysis and secondly developing novel models that can handle large quantities of data.Keywords: Healthcare analytics, predictive analytics, healthcare informatics, big data
1.1 Introduction
1.2 Big Data in Healthcare
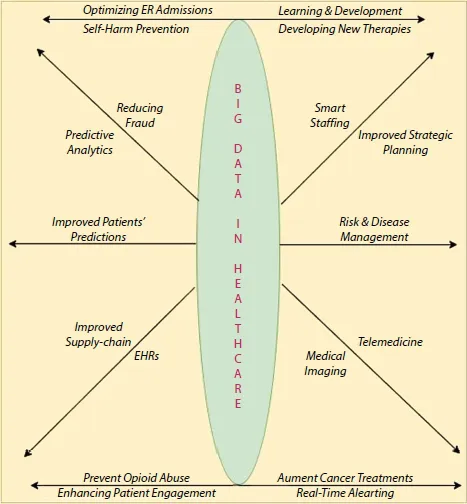
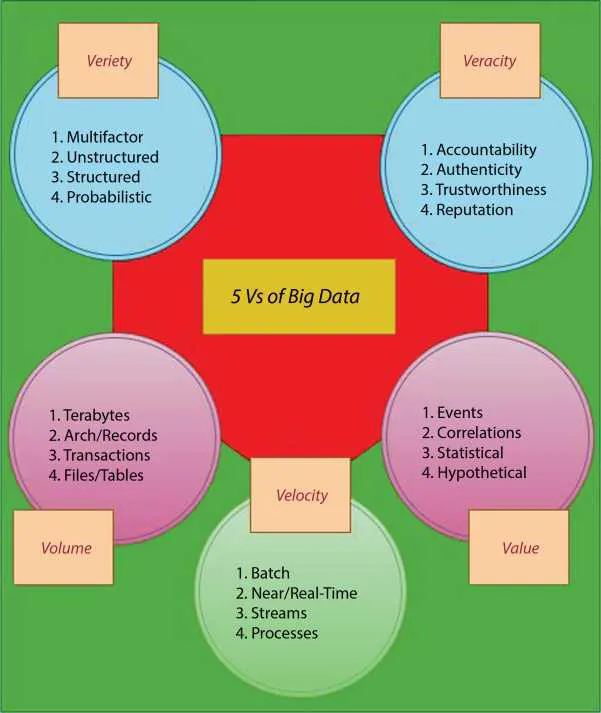
- 1. Variety: Without a doubt, the variety of data represents big data. For instance, among the various data formats are database, excel, and CSV, all of which can be stored in a plain text file. Additionally, structured, unstructured, and semi-structured health data exist. Clinical data is an example of structured information; however, unstructured or semi-structured data includes doctor notes, paper prescriptions, office medical records, images, and radio-graph films.
- 2. Veracity: This dataās legitimacy in the form of veracity can be challenged only if it is inaccurate. It is not about the accuracy of the data; it is about the capacity to process and interpretation of data. In healthcare, the trustworthiness function gives details on correct diagnosis, treatment, appropriate prescriptions, or otherwise established health outcomes.
- 3. Volume: Without a doubt, the large volume represents large amounts of data. To process massive amounts of data such as text, audio, video, and large-format images, existing data processing platforms and techniques must be strengthened. Personal information, radiology images, personal medical records, genomics, and biometric sensor readings, among other things, are gradually integrated into a healthcare database. All of this information adds significantly to the databaseās size and complexity.
- 4. Velocity: Big data is completely represented by the amount of information produced every second is considered as velocity. The information burst of social media has brought about a wide range of new and interesting data. Data on overall health condition and growth of the plant size and food bacteria are stored on paper, as well as various X-ray images and written reports, is up dramatically.
- 5. Value: Big data truly embodies the value of data. When it comes to big data analytics, the benefits and costs of analyzing and collecting big data are more important. In healthcare, the creation of value for patients should dictate how all other actors in the system are compensated. The primary goal of healthcare delivery must be to maximize value for patients.
1.3 Areas of Big Data Analytics in Medicine
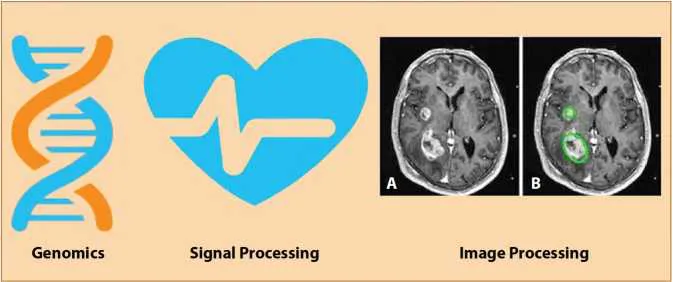