
eBook - ePub
Deep Learning in Biometrics
Mayank Vatsa, Richa Singh, Angshul Majumdar, Mayank Vatsa, Richa Singh, Angshul Majumdar
This is a test
Compartir libro
- 316 páginas
- English
- ePUB (apto para móviles)
- Disponible en iOS y Android
eBook - ePub
Deep Learning in Biometrics
Mayank Vatsa, Richa Singh, Angshul Majumdar, Mayank Vatsa, Richa Singh, Angshul Majumdar
Detalles del libro
Vista previa del libro
Índice
Citas
Información del libro
Deep Learning is now synonymous with applied machine learning. Many technology giants (e.g. Google, Microsoft, Apple, IBM) as well as start-ups are focusing on deep learning-based techniques for data analytics and artificial intelligence. This technology applies quite strongly to biometrics. This book covers topics in deep learning, namely convolutional neural networks, deep belief network and stacked autoencoders. The focus is also on the application of these techniques to various biometric modalities: face, iris, palmprint, and fingerprints, while examining the future trends in deep learning and biometric research.
-
- Contains chapters written by authors who are leading researchers in biometrics.
-
- Presents a comprehensive overview on the internal mechanisms of deep learning.
-
- Discusses the latest developments in biometric research.
-
- Examines future trends in deep learning and biometric research.
-
- Provides extensive references at the end of each chapter to enhance further study.
Preguntas frecuentes
¿Cómo cancelo mi suscripción?
¿Cómo descargo los libros?
Por el momento, todos nuestros libros ePub adaptables a dispositivos móviles se pueden descargar a través de la aplicación. La mayor parte de nuestros PDF también se puede descargar y ya estamos trabajando para que el resto también sea descargable. Obtén más información aquí.
¿En qué se diferencian los planes de precios?
Ambos planes te permiten acceder por completo a la biblioteca y a todas las funciones de Perlego. Las únicas diferencias son el precio y el período de suscripción: con el plan anual ahorrarás en torno a un 30 % en comparación con 12 meses de un plan mensual.
¿Qué es Perlego?
Somos un servicio de suscripción de libros de texto en línea que te permite acceder a toda una biblioteca en línea por menos de lo que cuesta un libro al mes. Con más de un millón de libros sobre más de 1000 categorías, ¡tenemos todo lo que necesitas! Obtén más información aquí.
¿Perlego ofrece la función de texto a voz?
Busca el símbolo de lectura en voz alta en tu próximo libro para ver si puedes escucharlo. La herramienta de lectura en voz alta lee el texto en voz alta por ti, resaltando el texto a medida que se lee. Puedes pausarla, acelerarla y ralentizarla. Obtén más información aquí.
¿Es Deep Learning in Biometrics un PDF/ePUB en línea?
Sí, puedes acceder a Deep Learning in Biometrics de Mayank Vatsa, Richa Singh, Angshul Majumdar, Mayank Vatsa, Richa Singh, Angshul Majumdar en formato PDF o ePUB, así como a otros libros populares de Informatique y Extraction de données. Tenemos más de un millón de libros disponibles en nuestro catálogo para que explores.
Información
1
Deep Learning: Fundamentals and Beyond
CONTENTS
1.1 Introduction
1.2 Restricted Boltzmann Machine
1.2.1 Incorporating supervision in RBMs
1.2.2 Other advances in RBMs
1.2.3 RBMs for biometrics
1.3 Autoencoder
1.3.1 Incorporating supervision in AEs
1.3.2 Other variations of AEs
1.4 Convolutional Neural Networks
1.4.1 Architecture of a traditional CNNs
1.4.2 Existing architectures of CNNs
1.5 Other Deep Learning Architectures
1.6 Deep Learning: Path Ahead
References
1.1 Introduction
The science of uniquely identifying a person based on his or her physiological or behavioral characteristics is termed biometrics. Physiological characteristics include face, iris, fingerprint, and DNA, whereas behavioral modalities include handwriting, gait, and keystroke dynamics. Jain et al. [1] lists seven factors that are essential for any trait (formally termed modality) to be used for biometric authentication. These factors are: universality, uniqueness, permanence, measurability, performance, acceptability, and circumvention.
An automated biometric system aims to either correctly predict the identity of the instance of a modality or verify whether the given sample is the same as the existing sample stored in the database. Figure 1.1 presents a traditional pipeline of a biometric authentication system. Input data corresponds to the raw data obtained directly from the sensor(s). Segmentation, or detection, refers to the process of extracting the region of interest from the given input. Once the required region of interest has been extracted, it is preprocessed to remove the noise, enhance the image, and normalize the data for subsequent ease of processing. After segmentation and preprocessing, the next step in the pipeline is feature extraction. Feature extraction refers to the process of extracting unique and discriminatory information from the given data. These features are then used for performing classification. Classification refers to the process of creating a model, which given a seen/unseen input feature vector is able to provide its correct label. For example, in case of a face recognition pipeline, the aim is to identify the individual in the given input sample. Here, the input data consists of images captured from the camera, containing at least one face image along with background or other objects. Segmentation, or detection, corresponds to detecting the face in the given input image. Several techniques can be applied for this step [2,3]; the most common being the Viola Jones face detector [4]. Once the faces are detected, they are normalized with respect to their geometry and intensity. For feature extraction, hand-crafted features such as Gabor filterbank [5], histogram of oriented gradients [6], and local binary patterns [7] and more recently, representation learning approaches have been used. The extracted features are then provided to a classifier such as a support vector machine [8] or random decision forest [9] for classification.

FIGURE 1.1
Illustrating the general biometrics authentication pipeline, which consists of five stages.
Illustrating the general biometrics authentication pipeline, which consists of five stages.
Automated biometric authentication systems have been used for several real-world applications, ranging from fingerprint sensors on mobile phones to border control applications at airports. One of the large-scale applications of automated biometric authentication is the ongoing project of Unique Identification Authority of India, pronounced “Aadhaar.” Initiated by the Indian Government,* the project aims to provide a unique identification number for each resident of India and capture his or her biometric modalities—face, fingerprint, and irises. This is done in an attempt to facilitate digital authentication anytime, anywhere, using the collected biometric data. Currently, the project has enrolled more than 1.1 billion individuals. Such large-scale projects often result in data having large intraclass variations, low interclass variations, and unconstrained environments.
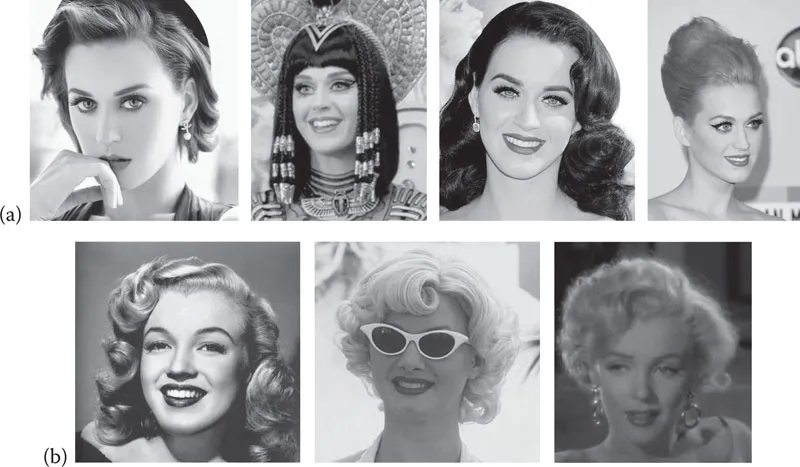
FIGURE 1.2
Sample images showcasing the large intraclass and low interclass variations that can be observed for the problem of face recognition. All images are taken from the Internet: (a) Images belonging to the same subject depicting high intraclass variations and (b) Images belonging to the different subjects showing low interclass variations. (Top, from left to right: https://tinyurl.com/y7hbvwsy,https://tinyurl.com/ydx3mvbf,https://tinyurl.com/y9uryu, https://tinyurl.com/y8lrnvrm; bottom from left to right, https://tinyurl.com/ybgvst84, https://tinyurl.com/y8762gl3, https://tinyurl.com/y956vrb6.)
Sample images showcasing the large intraclass and low interclass variations that can be observed for the problem of face recognition. All images are taken from the Internet: (a) Images belonging to the same subject depicting high intraclass variations and (b) Images belonging to the different subjects showing low interclass variations. (Top, from left to right: https://tinyurl.com/y7hbvwsy,https://tinyurl.com/ydx3mvbf,https://tinyurl.com/y9uryu, https://tinyurl.com/y8lrnvrm; bottom from left to right, https://tinyurl.com/ybgvst84, https://tinyurl.com/y8762gl3, https://tinyurl.com/y956vrb6.)
Figure 1.2 presents sample face images that illustrate the low interclass and high intraclass variations that can be observed in face recognition. In an attempt to model the challenges of real-world applications, several largescale data sets, such as MegaFace [10], CelebA [11], and CMU Multi-PIE [12] have been prepared. The availability of large data sets and sophisticated technologies (both hardware and algorithms) provide researchers the resources to model the variations observed in the data. These variations can be modeled in either of the four stages shown in Figure 1.1. Each of the four stages in the biometrics pipeline can also be viewed as separate machine learning tasks, which involve learning of the optimal parameters to enhance the final authentication performance. For instance, in the segmentation stage, each pixel can be classified into modality (foreground) or background [13,14]. Similarly, at the time of preprocessing, based on prior knowledge, different techniques can be applied depending on the type or quality of input [15]. Moreover, because of the progress in machine learning research, feature extraction is now viewed as a learning task.
Traditionally, research in feature extraction focused largely on handcrafted features such as Gabor and Haralick features [5,16], histogram of oriented gradients [6], and local binary patterns [7]. Many such hand-crafted features encode the pixel variations in the images to generate robust feature vectors for performing classification. Building on these, more complex hand-crafted features are also proposed that encode rotation and scale variations in the feature vectors as well [17,18]. With the availability of training data, researchers have started focusing on learning-based techniques, resulting in several representation learning-based algorithms. Moreover, because the premise is to train the machines for tasks performed with utmost ease by humans, it seemed fitting to understand and imitate the functioning of the human brain. This led researchers to reproduce similar structures to automate complex tasks, which gave rise to the dom...