
Statistics for Machine Learning
Pratap Dangeti
- 442 páginas
- English
- ePUB (apto para móviles)
- Disponible en iOS y Android
Statistics for Machine Learning
Pratap Dangeti
Información del libro
Build Machine Learning models with a sound statistical understanding.About This Book• Learn about the statistics behind powerful predictive models with p-value, ANOVA, and F- statistics.• Implement statistical computations programmatically for supervised and unsupervised learning through K-means clustering.• Master the statistical aspect of Machine Learning with the help of this example-rich guide to R and Python.Who This Book Is ForThis book is intended for developers with little to no background in statistics, who want to implement Machine Learning in their systems. Some programming knowledge in R or Python will be useful.What You Will Learn• Understand the Statistical and Machine Learning fundamentals necessary to build models• Understand the major differences and parallels between the statistical way and the Machine Learning way to solve problems• Learn how to prepare data and feed models by using the appropriate Machine Learning algorithms from the more-than-adequate R and Python packages• Analyze the results and tune the model appropriately to your own predictive goals• Understand the concepts of required statistics for Machine Learning• Introduce yourself to necessary fundamentals required for building supervised & unsupervised deep learning models• Learn reinforcement learning and its application in the field of artificial intelligence domainIn DetailComplex statistics in Machine Learning worry a lot of developers. Knowing statistics helps you build strong Machine Learning models that are optimized for a given problem statement. This book will teach you all it takes to perform complex statistical computations required for Machine Learning. You will gain information on statistics behind supervised learning, unsupervised learning, reinforcement learning, and more. Understand the real-world examples that discuss the statistical side of Machine Learning and familiarize yourself with it. You will also design programs for performing tasks such as model, parameter fitting, regression, classification, density collection, and more.By the end of the book, you will have mastered the required statistics for Machine Learning and will be able to apply your new skills to any sort of industry problem.Style and approachThis practical, step-by-step guide will give you an understanding of the Statistical and Machine Learning fundamentals you'll need to build models.
Preguntas frecuentes
Información
Unsupervised Learning
- Genomics: Unsupervised learning applied to understanding genomic-wide biological insights from DNA to better understand diseases and peoples. These types of tasks are more exploratory in nature.
- Search engine: Search engines might choose which search results to display to a particular individual based on the click histories of other similar users.
- Knowledge extraction: To extract the taxonomies of concepts from raw text to generate the knowledge graph to create the semantic structures in the field of NLP.
- Segmentation of customers: In the banking industry, unsupervised learning like clustering is applied to group similar customers, and based on those segments, marketing departments design their contact strategies. For example, older, low-risk customers will be targeted with fixed deposit products and high-risk, younger customers will be targeted with credit cards or mutual funds, and so on.
- Social network analysis: To identify the cohesive groups of people in social networks who are more connected with each other and have similar characteristics in common.
- K-means clustering
- Principal component analysis
- Singular value decomposition
- Deep auto encoders
K-means clustering
- In anti-money laundering measures, suspicious activities and individuals can be identified using anomaly detection
- In biology, clustering is used to find groups of genes with similar expression patterns
- In marketing analytics, clustering is used to find segments of similar customers so that different marketing strategies can be applied to different customer segments accordingly
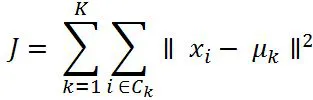