
Hands-On Transfer Learning with Python
Implement advanced deep learning and neural network models using TensorFlow and Keras
Dipanjan Sarkar, Raghav Bali, Tamoghna Ghosh
- 438 páginas
- English
- ePUB (apto para móviles)
- Disponible en iOS y Android
Hands-On Transfer Learning with Python
Implement advanced deep learning and neural network models using TensorFlow and Keras
Dipanjan Sarkar, Raghav Bali, Tamoghna Ghosh
Información del libro
Deep learning simplified by taking supervised, unsupervised, and reinforcement learning to the next level using the Python ecosystem
Key Features
- Build deep learning models with transfer learning principles in Python
- implement transfer learning to solve real-world research problems
- Perform complex operations such as image captioning neural style transfer
Book Description
Transfer learning is a machine learning (ML) technique where knowledge gained during training a set of problems can be used to solve other similar problems.
The purpose of this book is two-fold; firstly, we focus on detailed coverage of deep learning (DL) and transfer learning, comparing and contrasting the two with easy-to-follow concepts and examples. The second area of focus is real-world examples and research problems using TensorFlow, Keras, and the Python ecosystem with hands-on examples.
The book starts with the key essential concepts of ML and DL, followed by depiction and coverage of important DL architectures such as convolutional neural networks (CNNs), deep neural networks (DNNs), recurrent neural networks (RNNs), long short-term memory (LSTM), and capsule networks. Our focus then shifts to transfer learning concepts, such as model freezing, fine-tuning, pre-trained models including VGG, inception, ResNet, and how these systems perform better than DL models with practical examples. In the concluding chapters, we will focus on a multitude of real-world case studies and problems associated with areas such as computer vision, audio analysis and natural language processing (NLP).
By the end of this book, you will be able to implement both DL and transfer learning principles in your own systems.
What you will learn
- Set up your own DL environment with graphics processing unit (GPU) and Cloud support
- Delve into transfer learning principles with ML and DL models
- Explore various DL architectures, including CNN, LSTM, and capsule networks
- Learn about data and network representation and loss functions
- Get to grips with models and strategies in transfer learning
- Walk through potential challenges in building complex transfer learning models from scratch
- Explore real-world research problems related to computer vision and audio analysis
- Understand how transfer learning can be leveraged in NLP
Who this book is for
Hands-On Transfer Learning with Python is for data scientists, machine learning engineers, analysts and developers with an interest in data and applying state-of-the-art transfer learning methodologies to solve tough real-world problems. Basic proficiency in machine learning and Python is required.
Preguntas frecuentes
Información
Deep Learning Essentials
- What is deep learning?
- Deep learning fundamentals
- Setting up a robust, cloud-based deep learning environment with GPU support
- Setting up a robust, on-premise deep learning environment with GPU support
- Neural network basics
What is deep learning?
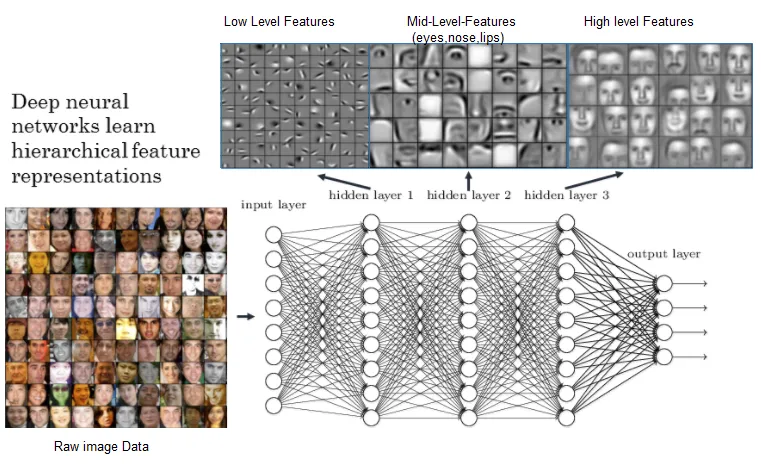