
Machine Learning Bookcamp
Build a portfolio of real-life projects
Alexey Grigorev
- 472 pagine
- English
- ePUB (disponibile sull'app)
- Disponibile su iOS e Android
Machine Learning Bookcamp
Build a portfolio of real-life projects
Alexey Grigorev
Informazioni sul libro
Time to flex your machine learning muscles! Take on the carefully designed challenges of the Machine Learning Bookcamp and master essential ML techniques through practical application. Summary
In Machine Learning Bookcamp you will: Collect and clean data for training models
Use popular Python tools, including NumPy, Scikit-Learn, and TensorFlow
Apply ML to complex datasets with images
Deploy ML models to a production-ready environment The only way to learn is to practice! In Machine Learning Bookcamp, you'll create and deploy Python-based machine learning models for a variety of increasingly challenging projects. Taking you from the basics of machine learning to complex applications such as image analysis, each new project builds on what you've learned in previous chapters. You'll build a portfolio of business-relevant machine learning projects that hiring managers will be excited to see. Purchase of the print book includes a free eBook in PDF, Kindle, and ePub formats from Manning Publications. About the technology
Master key machine learning concepts as you build actual projects! Machine learning is what you need for analyzing customer behavior, predicting price trends, evaluating risk, and much more. To master ML, you need great examples, clear explanations, and lots of practice. This book delivers all three! About the book
Machine Learning Bookcamp presents realistic, practical machine learning scenarios, along with crystal-clear coverage of key concepts. In it, you'll complete engaging projects, such as creating a car price predictor using linear regression and deploying a churn prediction service. You'll go beyond the algorithms and explore important techniques like deploying ML applications on serverless systems and serving models with Kubernetes and Kubeflow. Dig in, get your hands dirty, and have fun building your ML skills! What's inside Collect and clean data for training models
Use popular Python tools, including NumPy, Scikit-Learn, and TensorFlow
Deploy ML models to a production-ready environmentAbout the reader
Python programming skills assumed. No previous machine learning knowledge is required. About the author
Alexey Grigorev is a principal data scientist at OLX Group. He runs DataTalks.Club, a community of people who love data.Table of Contents 1 Introduction to machine learning
2 Machine learning for regression
3 Machine learning for classification
4 Evaluation metrics for classification
5 Deploying machine learning models
6 Decision trees and ensemble learning
7 Neural networks and deep learning
8 Serverless deep learning
9 Serving models with Kubernetes and Kubeflow
Domande frequenti
Informazioni
1 Introduction to machine learning
- Understanding machine learning and the problems it can solve
- Organizing a successful machine learning project
- Training and selecting machine learning models
- Performing model validation
1.1 Machine learning
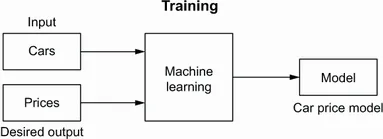
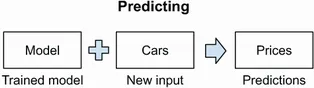
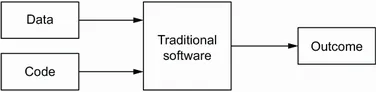
![]() | (A) In traditional software we discover patterns manually and encode them using a programming language. |
![]() | (B) A machine learning system discovers patterns automatically by learning from examples. After training, it produces a model that “knows” these patterns, but we still need to supervise it to make sure the model is correct. |
1.1.1 Machine learning vs. rule-based systems
- If sender = [email protected], then “spam”
- If title contains “buy now 50% off” and sender domain is “online.com,” then “spam”
- Otherwise, “good email”
- If sender = “[email protected]” then “spam”
- If title contains “buy now 50% off” and sender domain is “online.com,” then “spam”
- If body contains a word “deposit,” then “spam”
- Otherwise, “good email”