
How to Lead in Data Science
Jike Chong, Yue Cathy Chang
- 512 pagine
- English
- ePUB (disponibile sull'app)
- Disponibile su iOS e Android
How to Lead in Data Science
Jike Chong, Yue Cathy Chang
Informazioni sul libro
A field guide for the unique challenges of data science leadership, filled with transformative insights, personal experiences, and industry examples. In How To Lead in Data Science you will learn: Best practices for leading projects while balancing complex trade-offs
Specifying, prioritizing, and planning projects from vague requirements
Navigating structural challenges in your organization
Working through project failures with positivity and tenacity
Growing your team with coaching, mentoring, and advising
Crafting technology roadmaps and championing successful projects
Driving diversity, inclusion, and belonging within teams
Architecting a long-term business strategy and data roadmap as an executive
Delivering a data-driven culture and structuring productive data science organizations How to Lead in Data Science is full of techniques for leading data science at every seniority level—from heading up a single project to overseeing a whole company's data strategy. Authors Jike Chong and Yue Cathy Chang share hard-won advice that they've developed building data teams for LinkedIn, Acorns, Yiren Digital, large asset-management firms, Fortune 50 companies, and more. You'll find advice on plotting your long-term career advancement, as well as quick wins you can put into practice right away. Carefully crafted assessments and interview scenarios encourage introspection, reveal personal blind spots, and highlight development areas. About the technology
Lead your data science teams and projects to success! To make a consistent, meaningful impact as a data science leader, you must articulate technology roadmaps, plan effective project strategies, support diversity, and create a positive environment for professional growth. This book delivers the wisdom and practical skills you need to thrive as a data science leader at all levels, from team member to the C-suite. About the book
How to Lead in Data Science shares unique leadership techniques from high-performance data teams. It's filled with best practices for balancing project trade-offs and producing exceptional results, even when beginning with vague requirements or unclear expectations. You'll find a clearly presented modern leadership framework based on current case studies, with insights reaching all the way to Aristotle and Confucius. As you read, you'll build practical skills to grow and improve your team, your company's data culture, and yourself. What's inside How to coach and mentor team members
Navigate an organization's structural challenges
Secure commitments from other teams and partners
Stay current with the technology landscape
Advance your career About the reader
For data science practitioners at all levels. About the author
Dr. Jike Chong and Yue Cathy Chang build, lead, and grow high-performing data teams across industries in public and private companies, such as Acorns, LinkedIn, large asset-management firms, and Fortune 50 companies. Table of Contents
1 What makes a successful data scientist?
PART 1 THE TECH LEAD: CULTIVATING LEADERSHIP
2 Capabilities for leading projects
3 Virtues for leading projects
PART 2 THE MANAGER: NURTURING A TEAM
4 Capabilities for leading people
5 Virtues for leading people
PART 3 THE DIRECTOR: GOVERNING A FUNCTION
6 Capabilities for leading a function
7 Virtues for leading a function
PART 4 THE EXECUTIVE: INSPIRING AN INDUSTRY
8 Capabilities for leading a company
9 Virtues for leading a company
PART 5 THE LOOP AND THE FUTURE
10 Landscape, organization, opportunity, and practice
11 Leading in data science and a future outlook
Domande frequenti
Informazioni
1 What makes a successful data scientist?
- Learning what is expected of data scientists
- Examining the challenges of a data scientist’s career progression
![]() |
1.1 Data scientist expectations
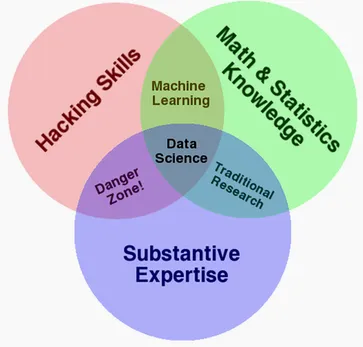