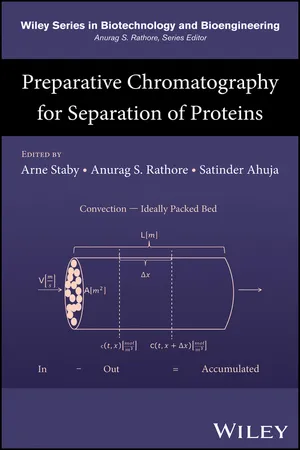
eBook - ePub
Preparative Chromatography for Separation of Proteins
Arne Staby, Anurag S. Rathore, Satinder Ahuja, Arne Staby, Anurag S. Rathore, Satinder Ahuja
This is a test
- English
- ePUB (disponibile sull'app)
- Disponibile su iOS e Android
eBook - ePub
Preparative Chromatography for Separation of Proteins
Arne Staby, Anurag S. Rathore, Satinder Ahuja, Arne Staby, Anurag S. Rathore, Satinder Ahuja
Dettagli del libro
Anteprima del libro
Indice dei contenuti
Citazioni
Informazioni sul libro
Preparative Chromatography for Separation of Proteins addresses a wide range of modeling, techniques, strategies, and case studies of industrial separation of proteins and peptides. • Covers broad aspects of preparative chromatography with a unique combination of academic and industrial perspectives
• Presents Combines modeling with compliantce useing of Quality-by-Design (QbD) approaches including modeling
• Features a variety of chromatographic case studies not readily accessible to the general public
• Represents an essential reference resource for academic, industrial, and pharmaceutical researchers
Domande frequenti
Come faccio ad annullare l'abbonamento?
È semplicissimo: basta accedere alla sezione Account nelle Impostazioni e cliccare su "Annulla abbonamento". Dopo la cancellazione, l'abbonamento rimarrà attivo per il periodo rimanente già pagato. Per maggiori informazioni, clicca qui
È possibile scaricare libri? Se sì, come?
Al momento è possibile scaricare tramite l'app tutti i nostri libri ePub mobile-friendly. Anche la maggior parte dei nostri PDF è scaricabile e stiamo lavorando per rendere disponibile quanto prima il download di tutti gli altri file. Per maggiori informazioni, clicca qui
Che differenza c'è tra i piani?
Entrambi i piani ti danno accesso illimitato alla libreria e a tutte le funzionalità di Perlego. Le uniche differenze sono il prezzo e il periodo di abbonamento: con il piano annuale risparmierai circa il 30% rispetto a 12 rate con quello mensile.
Cos'è Perlego?
Perlego è un servizio di abbonamento a testi accademici, che ti permette di accedere a un'intera libreria online a un prezzo inferiore rispetto a quello che pagheresti per acquistare un singolo libro al mese. Con oltre 1 milione di testi suddivisi in più di 1.000 categorie, troverai sicuramente ciò che fa per te! Per maggiori informazioni, clicca qui.
Perlego supporta la sintesi vocale?
Cerca l'icona Sintesi vocale nel prossimo libro che leggerai per verificare se è possibile riprodurre l'audio. Questo strumento permette di leggere il testo a voce alta, evidenziandolo man mano che la lettura procede. Puoi aumentare o diminuire la velocità della sintesi vocale, oppure sospendere la riproduzione. Per maggiori informazioni, clicca qui.
Preparative Chromatography for Separation of Proteins è disponibile online in formato PDF/ePub?
Sì, puoi accedere a Preparative Chromatography for Separation of Proteins di Arne Staby, Anurag S. Rathore, Satinder Ahuja, Arne Staby, Anurag S. Rathore, Satinder Ahuja in formato PDF e/o ePub, così come ad altri libri molto apprezzati nelle sezioni relative a Tecnologia e ingegneria e Ingegneria chimica e biochimica. Scopri oltre 1 milione di libri disponibili nel nostro catalogo.
Informazioni
1
Model‐Based Preparative Chromatography Process Development in the QbD Paradigm
Arne Staby1, Satinder Ahuja2, and Anurag S. Rathore3
1 CMC Project Planning & Management, Novo Nordisk A/S, Bagsværd, Denmark
2 Ahuja Consulting, Calabash, NC, USA
3 Department of Chemical Engineering, Indian Institute of Technology, New Delhi, India
1.1 Motivation
Preparative chromatography for separation of proteins and peptides continues to be the primary workhorse in purification of biopharmaceuticals. Numerous papers and books exist describing theory and implementation of preparative chromatography; however, this is the first book that combines academic progress in modeling with industrial implementation. Although theory and models have been available for many years, industrial usage of these tools has been scarce due to labor‐ and material‐intensive requirements. However, with the biotech industry moving to implement the expectations underlined in the recent regulatory initiative of quality by design (QbD), interesting and outspread applications of modeling tools for commercial process development and manufacture have emerged.
1.2 Regulatory Context of Preparative Chromatography and Process Understanding
QbD expectations to biopharmaceutical production including preparative chromatography are described in the ICH quality guidelines Q8, Q9, Q10, and Q11 [1–4]. Further, ICH Q8‐R2 [1] provides the overall definition of QbD in a regulatory context.
A systematic approach to development that begins with predefined objectives and emphasizes product and process understanding and process control based on sound science and quality risk management.
The focus of this book is on the underlined parts of this definition, and the framework of QbD may be outlined as presented in Figure 1.1. In the top part of the figure, the primary focus of biopharmaceuticals is the patient, and the patient needs are defined through the quality target product profile (QTPP), which in turn is affected by chemistry, manufacturing, and controls (CMC) activities. Fulfilling patients’ needs places some requirements on the product, and these elements are obtained through linkage of the QTPP to the list of critical quality attributes (CQAs). The CQAs will have acceptable ranges for the manufacturer to comply with, and to obtain product of the desired quality, the process needs to be run within acceptable ranges of process parameters. Proper knowledge of how process parameters affect the product quality may be obtained through process models that may end up in a regulatory, enhanced application for approval of a design space. To control process parameters within defined ranges, process models and/or even a design space will provide some requirements to the GMP facility and linkage to the control strategy, which will include various process monitors, process analytical technology (PAT) tools, process validation, and release tests and specifications. All elements are linked through risk assessment exercises to address the risk‐based approach of QbD in a regulatory setting.
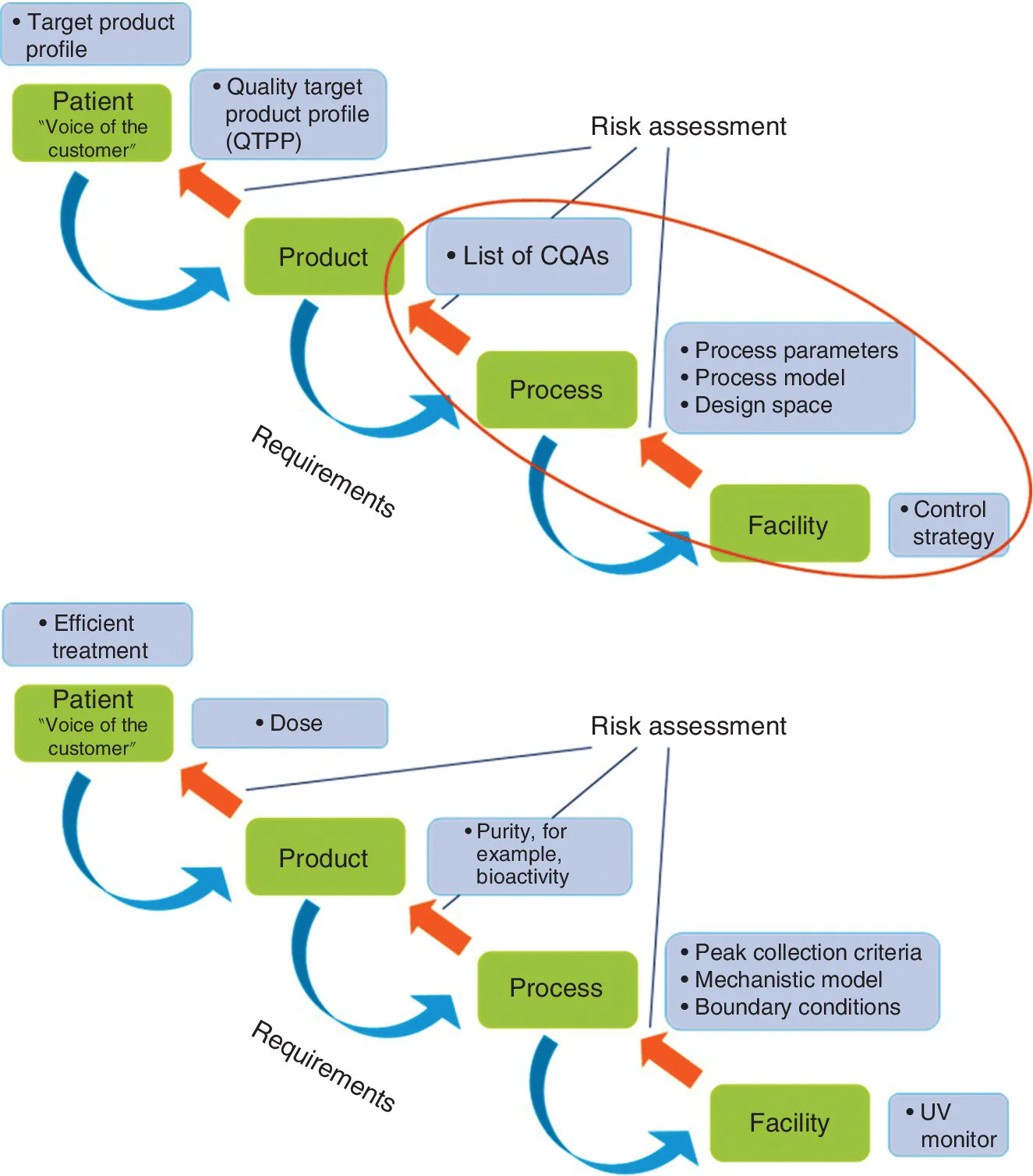
Figure 1.1 (Top) The framework of QbD. (Bottom) Example of QbD elements contained in the QbD framework for a preparative chromatography step.
Figure 1.1 (bottom) displays an example of QbD elements contained in the QbD framework for a preparative chromatography step. A key patient need is of course to get efficient treatment, and one element affecting this is to get a proper dose of the biopharmaceutical. To obtain proper dosing, the purity and among others the bioactivity of the biopharmaceutical needs to be correct. Purity is significantly affected by the peak collection criteria used in preparative chromatography, and a well‐known methodology for peak collection is by UV monitoring as part of the control strategy (e.g., see Chapters 12 and 17). A proper understanding and control of the preparative chromatography process may be obtained by a mechanistic or statistical model and their boundary conditions that may define an operational design space. Thus, the idea of this linkage exercise is to obtain a complete overview of the process in a way that will elucidate, for example, how a defect in or removal of a UV monitor in a preparative chromatographic purification step will affect the patient through cascading back in the figure through a series of risk assessments. The focus of this book is to obtain “process understanding and process control based on sound science” as described earlier, and it can be visualized by observing the elements within the red circle in Figure 1.1 (top).
A proper control strategy is achieved through sufficient process understanding. Traditionally, process understanding in the biopharmaceutical industry was obtained through a combination of theoretical knowledge based on the following: (i) education; (ii) experience from other projects and proteins optionally of similar nature, for example, mAbs; (iii) preliminary experimentation of less systematic nature; and (iv) “one parameter at a time” (OPAT) experimentation where all variables are kept constant while systematically altering one variable. This concept has worked well for many years, and most legacy products have been developed using this approach. Figure 1.2 presents the general level of knowledge obtained by the different methodologies including more recent concepts. Although some companies have also used multivariate methods for development and documentation of legacy products, the extensive use of more advanced methods for process understanding has been affected by implementation of QbD concepts. The general methodology used in the industry today is based on multivariate statistical analysis such as design of experiments (DoE) often combined with various high‐throughput process development (HTPD) techniques (see e.g., Chapter 11). DoE is a very broad and important tool that does not require mechanistic understanding prior to implementation, and it works quite efficiently if the user has prior knowledge of which parameters are significant and if the number of parameters is limited. Today, the most comprehensive application of statistical methods to support QbD and a true enhanced approach filing has been accomplished by Genentech/Roche with its recent regulatory approval of Gazyva. Disadvantages of DoE include less optimal identification of assumptions and the general lack of opportunities for extrapolation outside the experimental area used to set up the statistical models. DoE is used extensively for validation of parameter ranges in preparative chromatography; however for other unit operations such as fermentation, more advanced statistical methods like principal component analysis (PCA), partial least squares (PLS) methods, etc. are used due to their capability to handle very high number of variables (see also Chapter 16). At the top of the pyramid in Figure 1.2 and at the highest extent of knowledge obtainable are models based on mechanistic principles because full mechanistic process understanding is typically achieved. Depending on assumptions, these mechanistic models are also referred to as first‐principle models, and they provide...