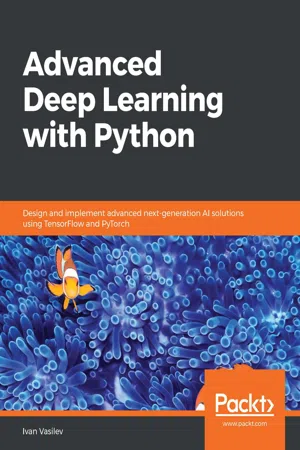
Advanced Deep Learning with Python
Design and implement advanced next-generation AI solutions using TensorFlow and PyTorch
Ivan Vasilev
- 468 pages
- English
- ePUB (mobile friendly)
- Available on iOS & Android
Advanced Deep Learning with Python
Design and implement advanced next-generation AI solutions using TensorFlow and PyTorch
Ivan Vasilev
About This Book
Gain expertise in advanced deep learning domains such as neural networks, meta-learning, graph neural networks, and memory augmented neural networks using the Python ecosystem
Key Features
- Get to grips with building faster and more robust deep learning architectures
- Investigate and train convolutional neural network (CNN) models with GPU-accelerated libraries such as TensorFlow and PyTorch
- Apply deep neural networks (DNNs) to computer vision problems, NLP, and GANs
Book Description
In order to build robust deep learning systems, you'll need to understand everything from how neural networks work to training CNN models. In this book, you'll discover newly developed deep learning models, methodologies used in the domain, and their implementation based on areas of application.
You'll start by understanding the building blocks and the math behind neural networks, and then move on to CNNs and their advanced applications in computer vision. You'll also learn to apply the most popular CNN architectures in object detection and image segmentation. Further on, you'll focus on variational autoencoders and GANs. You'll then use neural networks to extract sophisticated vector representations of words, before going on to cover various types of recurrent networks, such as LSTM and GRU. You'll even explore the attention mechanism to process sequential data without the help of recurrent neural networks (RNNs). Later, you'll use graph neural networks for processing structured data, along with covering meta-learning, which allows you to train neural networks with fewer training samples. Finally, you'll understand how to apply deep learning to autonomous vehicles.
By the end of this book, you'll have mastered key deep learning concepts and the different applications of deep learning models in the real world.
What you will learn
- Cover advanced and state-of-the-art neural network architectures
- Understand the theory and math behind neural networks
- Train DNNs and apply them to modern deep learning problems
- Use CNNs for object detection and image segmentation
- Implement generative adversarial networks (GANs) and variational autoencoders to generate new images
- Solve natural language processing (NLP) tasks, such as machine translation, using sequence-to-sequence models
- Understand DL techniques, such as meta-learning and graph neural networks
Who this book is for
This book is for data scientists, deep learning engineers and researchers, and AI developers who want to further their knowledge of deep learning and build innovative and unique deep learning projects. Anyone looking to get to grips with advanced use cases and methodologies adopted in the deep learning domain using real-world examples will also find this book useful. Basic understanding of deep learning concepts and working knowledge of the Python programming language is assumed.
Frequently asked questions
Information
Section 1: Core Concepts
- Chapter 1, The Nuts and Bolts of Neural Networks
The Nuts and Bolts of Neural Networks
- The mathematical apparatus of NNs
- A short introduction to NNs
- Training NNs
The mathematical apparatus of NNs
Linear algebra

- Scalars: A single number.
- Vectors: A one-dimensional array of numbers (or components). Each component of the array has an index. In literature, we will see vectors denoted either with a superscript arrow (

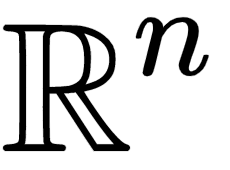
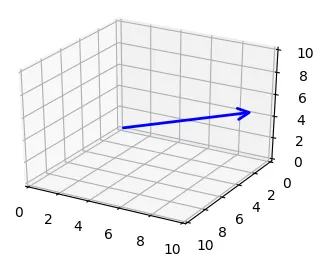
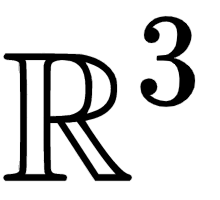
- Magnitude (or length) is a generalization of the Pythagorean theorem for an n-dimensional space:

- Direction is the angle of the vector along each axis of the vector space.
- Matrices: This is a two-dimensional array of numbers. Each element is identified by two indices (row and column). A matrix is usually denoted with a bold capital letter; for example...