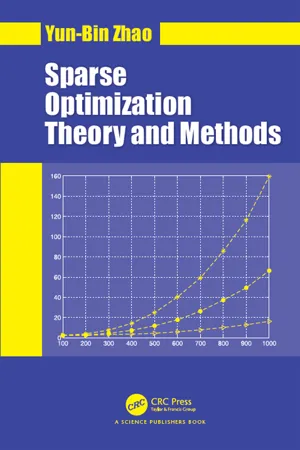
Sparse Optimization Theory and Methods
Yun-Bin Zhao
- 284 pages
- English
- ePUB (mobile friendly)
- Available on iOS & Android
Sparse Optimization Theory and Methods
Yun-Bin Zhao
About This Book
Seeking sparse solutions of underdetermined linear systems is required in many areas of engineering and science such as signal and image processing. The efficient sparse representation becomes central in various big or high-dimensional data processing, yielding fruitful theoretical and realistic results in these fields. The mathematical optimization plays a fundamentally important role in the development of these results and acts as the mainstream numerical algorithms for the sparsity-seeking problems arising from big-data processing, compressed sensing, statistical learning, computer vision, and so on. This has attracted the interest of many researchers at the interface of engineering, mathematics and computer science.
Sparse Optimization Theory and Methods presents the state of the art in theory and algorithms for signal recovery under the sparsity assumption. The up-to-date uniqueness conditions for the sparsest solution of underdertemined linear systems are described. The results for sparse signal recovery under the matrix property called range space property (RSP) are introduced, which is a deep and mild condition for the sparse signal to be recovered by convex optimization methods. This framework is generalized to 1-bit compressed sensing, leading to a novel sign recovery theory in this area. Two efficient sparsity-seeking algorithms, reweighted l1-minimization in primal space and the algorithm based on complementary slackness property, are presented. The theoretical efficiency of these algorithms is rigorously analysed in this book. Under the RSP assumption, the author also provides a novel and unified stability analysis for several popular optimization methods for sparse signal recovery, including l1-mininization, Dantzig selector and LASSO. This book incorporates recent development and the author's latest research in the field that have not appeared in other books.