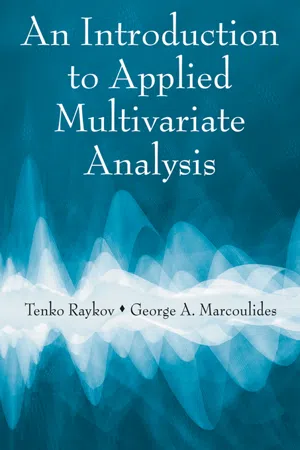
eBook - ePub
An Introduction to Applied Multivariate Analysis
Tenko Raykov, George A. Marcoulides
This is a test
- 496 pages
- English
- ePUB (mobile friendly)
- Available on iOS & Android
eBook - ePub
An Introduction to Applied Multivariate Analysis
Tenko Raykov, George A. Marcoulides
Book details
Book preview
Table of contents
Citations
About This Book
This comprehensive text introduces readers to the most commonly used multivariate techniques at an introductory, non-technical level. By focusing on the fundamentals, readers are better prepared for more advanced applied pursuits, particularly on topics that are most critical to the behavioral, social, and educational sciences. Analogies betwe
Frequently asked questions
How do I cancel my subscription?
Can/how do I download books?
At the moment all of our mobile-responsive ePub books are available to download via the app. Most of our PDFs are also available to download and we're working on making the final remaining ones downloadable now. Learn more here.
What is the difference between the pricing plans?
Both plans give you full access to the library and all of Perlegoâs features. The only differences are the price and subscription period: With the annual plan youâll save around 30% compared to 12 months on the monthly plan.
What is Perlego?
We are an online textbook subscription service, where you can get access to an entire online library for less than the price of a single book per month. With over 1 million books across 1000+ topics, weâve got you covered! Learn more here.
Do you support text-to-speech?
Look out for the read-aloud symbol on your next book to see if you can listen to it. The read-aloud tool reads text aloud for you, highlighting the text as it is being read. You can pause it, speed it up and slow it down. Learn more here.
Is An Introduction to Applied Multivariate Analysis an online PDF/ePUB?
Yes, you can access An Introduction to Applied Multivariate Analysis by Tenko Raykov, George A. Marcoulides in PDF and/or ePUB format, as well as other popular books in Economics & Statistics for Business & Economics. We have over one million books available in our catalogue for you to explore.
1
Introduction to Multivariate Statistics
One of the simplest conceptual definitions of multivariate statistics (MVS) is as a set of methods that deal with the simultaneous analysis of multiple outcome or response variables, frequently also referred to as dependent variables (DVs). This definition of MVS suggests an important relationship to univariate statistics (UVS) that may be considered a group of methods dealing with the analysis of a single DV. In fact, MVS not only exhibits similarities with UVS but can also be considered an extension of it, or conversely UVS can be viewed as a special case of MVS. At the same time, MVS and UVS have a number of distinctions, and this book deals with many of them whenever appropriate.
In this introductory chapter, our main objective is to discuss, from a principled standpoint, some of the similarities and differences between MVS and UVS. More specifically, we (a) define MVS; then (b) discuss some relationships between MVS and UVS; and finally (c) illustrate the use of the popular statistical software SPSS and SAS for a number of initial multiple variable analyses, including obtaining covariance, correlation, and sum-of-squares and cross-product matrices. As will be observed repeatedly throughout the book, these are three matrices of variable interrelationship indices, which play a fundamental role in many MVS methods.
1.1 Definition of Multivariate Statistics
Behavioral, social, and educational phenomena are often multifaceted, multifactorially determined, and exceedingly complex. Any systematic attempt to understand them, therefore, will typically require the examination of multiple dimensions that are usually intertwined in complicated ways. For these reasons, researchers need to evaluate a number of interrelated variables capturing specific aspects of phenomena under consideration. As a result, scholars in these sciences commonly obtain and have to deal with data sets that contain measurements on many interdependent dimensions, which are collected on subjects sampled from the studied populations. Consequently, in empirical behavioral and social studies, one is very often faced with data sets consisting of multiple interrelated variables that have been observed on a number of persons, and possibly on samples from different populations.
MVS is a scientific field, which for many purposes may be viewed a branch of mathematics and has been developed to meet these complex challenges. Specifically, MVS represents a multitude of statistical methods to help one analyze potentially numerous interrelated measures considered together rather than separately from one another (i.e., one at a time). Researchers typically resort to using MVS methods when they need to analyze more than one dependent (response, or outcome) variable, possibly along with one or more independent (predictor, or explanatory) variables, which are in general all correlated with each other.
Although the concepts of independent variables (IVs) and DVs are generally well covered in most introductory statistics and research methods treatments, for the aims of this chapter, we deem it useful to briefly discuss them here. IVs are typically different conditions to which subjects might be exposed, or reflect specific characteristics that studied persons bring into a research situation. For example, socioeconomic status (SES), educational level, age, gender, teaching method, training program or treatment are oftentimes considered IVs in various empirical settings. Conversely, DVs are those that are of main interest in an investigation, and whose examination is of focal interest to the researcher. For example, intelligence, aggression, college grade point average (GPA) or Graduate Record Exam (GRE) score, performance on a reading or writing test, math ability score or computer aptitude score can be DVs in a study aimed at explaining variability in any of these measures in terms of some selected IVs. More specifically, the IVs and DVs are defined according to the research question being asked. For this reason, it is possible that a variable that is an IV for one research query may become a DV for another one, or vice versa. Even within a single study, it is not unlikely that a DV for one question of interest changes status to an IV, or conversely, when pursuing another concern at a different point during the study.
To give an example involving IVs and DVs: suppose an educational scientist were interested in comparing the effectiveness of two teaching methods, a standard method and a new method of teaching number division. To this end, two groups of students are randomly assigned to the new and to the standard method. Assume that a test of number division ability was administered to all students who participated in the study, and that the researcher was interested in explaining the individual differences observed then. In this case, the score on the division test would be a DV. If the scientist had measured initial arithmetic ability as well as collected data on student SES or even hours watching television per week then all these three variables may be potential IVs. The particular posited question appears to be relatively simple and in fact may be addressed straightforwardly using UVS as it is phrased in terms of a single DV, namely score obtained on the number division test. However, if the study was carried out in such a way that measurements of division ability were collected for each student on each of three consecutive weeks after the two teaching methods were administered, and in addition data on hours watched television were gathered in each of these weeks, then this question becomes considerably more complicated. This is because there are now three measures of interestâdivision ability in each of the 3 weeks of measurementâand it may be appropriate to consider them all as DVs. Furthermore, because these measures are taken on the same subjects in the study, they are typically interrelated. In addition, when addressing the original question about comparative effectiveness of the new teaching method relative to the old one, it would make sense at least in some analyses to consider all three so-obtained division ability scores simultaneously. Under these circumstances, UVS cannot provide the sought answer to the research query. This is when MVS is typically used, especially where the goal is to address complicated research questions that cannot be answered directly with UVS.
A main reason for this MVS preference in such situations is that UVS represents a set of statistical methods to deal with just a single DV, while there is effectively no limit on the number of IVs that might be considered. As an example, consider the question of whether observed individual differences in average university freshman grades could be explained with such on their SAT score. In this case, the DV is freshman GPA, while the SAT score would play the role of an IV, possibly in addition to say gender, SES and type of high school attended (e.g., public vs. private), in case a pursued research question requires consideration of these as further IVs.
There are many UVS and closely related methods that can be used to address an array of queries varying in their similarity to this one. For example, for prediction goals, one could consider using regression analysis (simple or multiple regression, depending on the number of IVs selected), including the familiar t test. When examination of mean differences across groups is of interest, a traditionally utilized method would be analysis of variance (ANOVA) as well as analysis of covariance (ANCOVA)âeither approach being a special case of regression analysis. Depending on the nature of available data, one may consider a chi-square analysis of say two-way frequency tables (e.g., for testing association of two categorical variables). When certain assumptions are markedly violated, in particular the assumption of normality, and depending on other study aspects, one may also consider nonparametric statistical methods. Most of the latter methods share the common feature that they are typically considered for application when for certain research questions one identifies single DVs.
By way of contrast, MVS may be viewed as an extension of UVS in the case where one is interested in studying multiple DVs that are interrelated, as they commonly would be in empirical research in the behavioral, social, and educational disciplines. For this reason, MVS typically deals in applications with fairly large data sets on potentially many subjects and in particular on possibly numerous interrelated variables of main interest. Due to this complexity, the underlying theme behind many MVS methods is also simplification, for instance, the reduction of the complexity of available data to several meaningful indices, quantities (parameters), or dimensions.
To give merely a sense of the range of questions addressed with MVS, let us consider a few simple examplesâwe of course return to these issues in greater detail later in this book. Suppose a researcher is interested in determining which characteristics or variables differentiate between achievers and nonachievers in an educational setting. As will be discussed in Chapter 10, these kinds of questions can be answered using a technique called discriminant function analysis (or discriminant analysis for short). Interestingly, discriminant analysis can also be used to predict group membershipâin the currently considered example, achievers versus nonachieversâbased on the knowledge obtained about differentiating characteristics or variables. Another research question may be whether there is a single underlying (i.e., unobservable, or so-called latent) dimension along which students differ and which is responsible for their observed interrelationships on some battery of intelligence tests. Such a question can be attended to using a method called factor analysis (FA), discussed in Chapters 8 and 9. As another example, one may be concerned with finding out whether a set of interrelated tests can be decomposed into groups of measures and accordingly new derived measures obtained so that they account for most of the observed variance of the tests. For these aims, a method called principal component analysis (PCA) is appropriate, to which we turn in Chapter 7. Fur...
Table of contents
Citation styles for An Introduction to Applied Multivariate Analysis
APA 6 Citation
Raykov, T., & Marcoulides, G. (2008). An Introduction to Applied Multivariate Analysis (1st ed.). Taylor and Francis. Retrieved from https://www.perlego.com/book/1519022/an-introduction-to-applied-multivariate-analysis-pdf (Original work published 2008)
Chicago Citation
Raykov, Tenko, and George Marcoulides. (2008) 2008. An Introduction to Applied Multivariate Analysis. 1st ed. Taylor and Francis. https://www.perlego.com/book/1519022/an-introduction-to-applied-multivariate-analysis-pdf.
Harvard Citation
Raykov, T. and Marcoulides, G. (2008) An Introduction to Applied Multivariate Analysis. 1st edn. Taylor and Francis. Available at: https://www.perlego.com/book/1519022/an-introduction-to-applied-multivariate-analysis-pdf (Accessed: 14 October 2022).
MLA 7 Citation
Raykov, Tenko, and George Marcoulides. An Introduction to Applied Multivariate Analysis. 1st ed. Taylor and Francis, 2008. Web. 14 Oct. 2022.