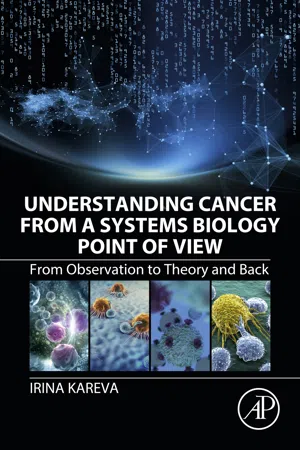
eBook - ePub
Understanding Cancer from a Systems Biology Point of View
From Observation to Theory and Back
Irina Kareva
This is a test
- 118 pages
- English
- ePUB (mobile friendly)
- Available on iOS & Android
eBook - ePub
Understanding Cancer from a Systems Biology Point of View
From Observation to Theory and Back
Irina Kareva
Book details
Book preview
Table of contents
Citations
About This Book
Understanding Cancer from a Systems Biology Point of View: From Observation to Theory and Back starts with a basic question, why do we sometimes observe accelerated metastatic growth after resection of primary tumors? Next, it helps readers understand the systemic nature of cancer and how it affects treatment approaches and decisions. The book puts together aspects of cancer that many readers have most likely never combined, using unfamiliar, novel methods. It is a valuable resource for cancer researchers, cancer biologists, mathematicians and members of the biomedical field who are interested in applying systems biology methodologies for understanding and treating cancer.
- Explains the systemic nature of cancer and how it affects decisions on treatment
- Brings a variety of methods together, showing, in detail, the logical approach to finding answers to complex questions
- Discusses the theoretical underpinnings of cancer as a systemic disease, providing the reader with valuable information on applicable cases
Frequently asked questions
How do I cancel my subscription?
Can/how do I download books?
At the moment all of our mobile-responsive ePub books are available to download via the app. Most of our PDFs are also available to download and we're working on making the final remaining ones downloadable now. Learn more here.
What is the difference between the pricing plans?
Both plans give you full access to the library and all of Perlegoās features. The only differences are the price and subscription period: With the annual plan youāll save around 30% compared to 12 months on the monthly plan.
What is Perlego?
We are an online textbook subscription service, where you can get access to an entire online library for less than the price of a single book per month. With over 1 million books across 1000+ topics, weāve got you covered! Learn more here.
Do you support text-to-speech?
Look out for the read-aloud symbol on your next book to see if you can listen to it. The read-aloud tool reads text aloud for you, highlighting the text as it is being read. You can pause it, speed it up and slow it down. Learn more here.
Is Understanding Cancer from a Systems Biology Point of View an online PDF/ePUB?
Yes, you can access Understanding Cancer from a Systems Biology Point of View by Irina Kareva in PDF and/or ePUB format, as well as other popular books in Medicine & Medical Theory, Practice & Reference. We have over one million books available in our catalogue for you to explore.
Information
Topic
MedicineChapter 1
Introduction
Research is driven by the interplay of theory and experiment. Experiments give rise to theories, which we test using experiments, which drive theory, until we find answers to questions that interest us. After all, research is first and foremost about satisfying scientific curiosity.
Some experiments are easy to run. Most are not. Experiments require planning, and for most biological questions, expensive equipment, laboratory space, and abundant resources. Even more importantly, investigating any biological system means trying to unravel the interplay of numerous factors, the influence of which on the final outcome is not always easy to tease out. That is where mathematical modeling can come in very handy.
The schematic approach for using mathematical modeling for testing hypotheses about biological mechanisms can be summarized as follows:
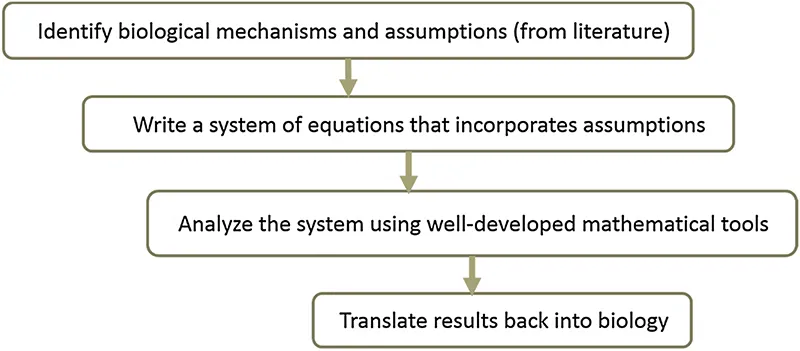
In a way, mathematical modeling is like Rosetta Stone, a way to translate back and forth between mathematics and biology. As modelers, we try to (1) understand a biological mechanism, (2) tease out key players that drive the dynamics, (3) formulate assumptions about how these key players interact with each other and their environment, (4) translate these assumptions into equations, (5) analyze them using a very well-developed mathematical apparatus, and (6) translate the results back into the language of biology. If our predictions are consistent with experimental observations, then maybe we got it right, and we can now try to make further predictions about what will happen if we change this or that aspect of the system. Conducting early in silico experiments can save thousands of dollars when planning further experiments, advancing a āfiscally responsibleā way of conducting research.
And what if the predictions made by the model are not consistent with experimental observations? That may be even more interesting. This means that some of our assumptions are incorrect, and thus our understanding of biology is incomplete. The good news is that since we built the model, we control all the assumptions, and so we can go through them one by one and identify which one is causing the discrepancy. Chances are we just identified a gap in knowledge, which we can now try to close using both theory and experiment.
However, all of this matters only when there is a question. A question that drives your curiosity, a question that bugs you like a pebble in your shoe. The question driving the work that led to this book stems from the following observation: sometimes after removal of primary tumors, metastases start growing faster. This has been observed particularly in colorectal cancer but in other cancer types as well. And the question is Why? What is going on?
It is possible that removing a primary tumor can result in some kind of malignant transformation, causing secondary tumors to form and rapidly start growing, but it is not very likely. Although some aggressive tumor types do exist, tumors typically take years and sometimes decades to form and grow. It is more likely that secondary tumors were there all along, either kept at bay or āsleeping,ā and something either about the process of primary tumor removal, such as surgery, or the changes that happen in the body after the removal of the primary tumor āwoke them up.ā Cancers that are present but do not grow are known as dormant tumors, cancer without disease. Trying to understand mechanisms that underlie the state of dormancy might hold a key to answering the question underlying this book.
However, before we dive into that, it is important to gain some understanding about the basic mechanisms of mathematical modeling.
A Primer on Mathematical Modeling: PredatorāPrey
A key aspect of understanding dynamical systems and writing mathematical models lies in thinking not of what things are but of what they do. This translates into thinking about relationships between individuals (people, animals, or cells), and how they interact with each other and their environment.
For instance, predatorāprey type models have been developed extensively in the literature, starting from the classic LotkaāVolterra models that describe the dynamics of two populations, where the growth of one is dependent on consuming the other. These models were used to explain the dynamics of natural populations of lynx and snowshoe hare in Canada, for instance. However, predatorāprey type models are also the classic framework for describing tumorāimmune interactions, where tumor is the āpreyā and immune system is āpredator.ā We will use this framework as an example for describing how to write a system of ordinary differential equations, which will be used in later chapters.
Equations describing tumor growth typically have the following components:
- ⢠Growth terms, often logistic or āGompertz,ā of which logistic form is a special case
- ⢠The carrying capacity (maximum population size that can be supported indefinitely) may remain fixed or can be dynamic, depending on the type of question the authors may be asking
- Death terms:
- ⢠Death due to apoptosis or preprogrammed natural death (this can be included in logistic growth or as a separate term)
- ⢠Death due to predation by the immune system
- ⢠Death due to the activity of a drug or chemical agent
Equations describing the dynamics of the immune cells typically focus on CD8+T cells (white blood cells that have originated in the thymus and that express the CD8 proteins on cell surface) as the most efficient cytotoxic predators; however, they can be expanded to include antigen presenting cells (APCs), natural killer (NK) cells, etc., depending on the questions and the level of detail that is necessary to answer them. Notably, increasing the level of detail is not necessarily beneficial and can make it more difficult to discern the effects of the processes investigated. Therefore, the level of detail should be driven by the question, and only cell types and agents that quantitatively affect the dynamics, directly or indirectly, should be taken into account.
Equations describing the dynamics of immune cells typically have the following components:
- Growth terms:
- ⢠Natural inflow (population growth) rates to describe baseline levels of the immune cells.
- ⢠Additional inflow of immune cells due to the presence of the tumor. Since the immune cells react to the antigens/debris of the previously killed tumor cells, this term is proportional to the number of previously killed tumor cells. This is an important difference of tumorāimmune models as compared to classic predatorāprey type models, where increase of the predator would be directly proportional to how much prey it has consumed, as opposed to how many ācarcassesā the predator has encountered.
- ⢠In some variations, there may be other factors contributing to the ability of the predator (immune system) to grow. For instance, in Chapter 3 on tumorāimmune interactions, we looked at the situation where both tumor and immune cells compete for glucose, a shared resource that is necessary for both populations to grow. Therefore, in this model, the term describing immune cell growth was also dependent on the level of available glucose.
- Death terms:
- ⢠There often exists a natural death term for the tumor-specific immune cells to indicate decrease in immune cell levels following decrease in tumor levels.
- ⢠There can also be a treatment-induced death term, which accounts for immune cell sensitivity to many cytotoxic drugs.
Once written, a system of differential equations is coded into a numerical solver, such as Matlab, and investigated numerically (in Matlab it is typically done using functions ode45 or ode23). To conduct a numerical investigation of a dynamical system, we identify parameters of interest for the question (this can be done based on understanding of the biology or through conducting sensitivity analysis, which will be discussed in later chapters), vary them, and create plots of how predicted population sizes change over time. This approach provides insights into what processes may be driving the dynamics of the investigated system, how different components of the system affect each other, and what may or may not be reasonable to try and reproduce in an experimental setting. Numerous examples will be given later in the book showing how this process is done.
Estimating Parameters
Many models appear to be overparameterized. As a rule, there should be as many parameters as are necessary for the units to balance out on both sides of the equation. That is, if we are tracking rate of change of population size over time on the left-hand side of the equation, we might have to introduce scaling parameters in the right-hand side terms to ensure balanced units.
Estimating parameter values for simulations may prove challenging because it may not always be possible to find experiments that will allow discerning exact values. Furthermore, in experiments, we may find that several parameters are combined into one, and discerning individual values for each may not always be possible or useful.
However, even in the absence of exact parameter values, it is important to be able to estimate the order of magnitude of the parameter value due to potential differences in time scales. If one of the described processes occurs qualitatively faster or slower than others, then failing to take this into account would attribute importance to a process that may not actually be affecting the dynamics, thus leading to incorrect or misleading predictions.
If one has access to experimental data, it may be possible to find parameters that allow reproducing the data using parameter fitting approaches such as least squares, Monte Carlo simulations, or built-in Matlab functions such as fmincon and fminsearch. This is also one of the best ways to validate the model before proceeding with further investigati...
Table of contents
Citation styles for Understanding Cancer from a Systems Biology Point of View
APA 6 Citation
Kareva, I. (2018). Understanding Cancer from a Systems Biology Point of View ([edition unavailable]). Elsevier Science. Retrieved from https://www.perlego.com/book/1829176/understanding-cancer-from-a-systems-biology-point-of-view-from-observation-to-theory-and-back-pdf (Original work published 2018)
Chicago Citation
Kareva, Irina. (2018) 2018. Understanding Cancer from a Systems Biology Point of View. [Edition unavailable]. Elsevier Science. https://www.perlego.com/book/1829176/understanding-cancer-from-a-systems-biology-point-of-view-from-observation-to-theory-and-back-pdf.
Harvard Citation
Kareva, I. (2018) Understanding Cancer from a Systems Biology Point of View. [edition unavailable]. Elsevier Science. Available at: https://www.perlego.com/book/1829176/understanding-cancer-from-a-systems-biology-point-of-view-from-observation-to-theory-and-back-pdf (Accessed: 15 October 2022).
MLA 7 Citation
Kareva, Irina. Understanding Cancer from a Systems Biology Point of View. [edition unavailable]. Elsevier Science, 2018. Web. 15 Oct. 2022.