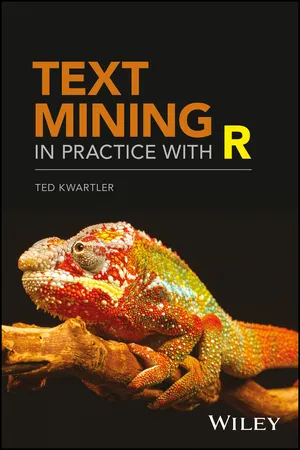
Text Mining in Practice with R
Ted Kwartler
- English
- ePUB (mobile friendly)
- Available on iOS & Android
Text Mining in Practice with R
Ted Kwartler
About This Book
A reliable, cost-effective approach to extracting priceless business information from all sources of text
Excavating actionable business insights from data is a complex undertaking, and that complexity is magnified by an order of magnitude when the focus is on documents and other text information. This book takes a practical, hands-on approach to teaching you a reliable, cost-effective approach to mining the vast, untold riches buried within all forms of text using R.
Author Ted Kwartler clearly describes all of the tools needed to perform text mining and shows you how to use them to identify practical business applications to get your creative text mining efforts started right away. With the help of numerous real-world examples and case studies from industries ranging from healthcare to entertainment to telecommunications, he demonstrates how to execute an array of text mining processes and functions, including sentiment scoring, topic modelling, predictive modelling, extracting clickbait from headlines, and more. You'll learn how to:
- Identify actionable social media posts to improve customer service
- Use text mining in HR to identify candidate perceptions of an organisation, match job descriptions with resumes, and more
- Extract priceless information from virtually all digital and print sources, including the news media, social media sites, PDFs, and even JPEG and GIF image files
- Make text mining an integral component of marketing in order to identify brand evangelists, impact customer propensity modelling, and much more
Most companies' data mining efforts focus almost exclusively on numerical and categorical data, while text remains a largely untapped resource. Especially in a global marketplace where being first to identify and respond to customer needs and expectations imparts an unbeatable competitive advantage, text represents a source of immense potential value. Unfortunately, there is no reliable, cost-effective technology for extracting analytical insights from the huge and ever-growing volume of text available online and other digital sources, as well as from paper documents—until now.
Frequently asked questions
Information
Chapter 1
What is Text Mining?
- the basic definition of practical text mining
- why text mining is important to the modern enterprise
- examples of text mining used in enterprise
- the challenges facing text mining
- an example workflow for processing natural language in analytical contexts
- a simple text mining example
- when text mining is appropriate
1.1 What is it?
1.1.1 What is Text Mining in Practice?
1.1.2 Where Does Text Mining Fit?
1.2 Why We Care About Text Mining
- Social media continues to evolve and affect an organization's public efforts.
- Online content from an organization, its competitors and outside sources, such as blogs, continues to grow.
- The digitization of formerly paper records is occurring in many legacy industries, such as healthcare.
- New technologies like automatic audio transcription are helping to capture customer touchpoints.
- As textual sources grow in quantity, complexity and number of sources, the concurrent advance in processing power and storage has translated to vast amounts of text being stored throughout an enterprise's data lake.
1.2.1 What Are the Consequences of Ignoring Text?
- Ignoring text is not an adequate response of an analytical endeavor. Rigorous scientific and analytical exploration requires investigating sources of information that can explain phenomena.
- Not performing text mining may lead an analysis to a false outcome.
- Some problems are almost entirely text-based, so not using these methods would mean significant reduction in effectiveness or even not being able to perform the analysis.
Wisdom of Crowds 1.1
mturk
or crowdflower
. Often communication theory does not support these methods as a sound way to score text, or to extract meaning. Setting aside sampling biases and logistical tabulation difficulties, communication theory states that the meaning of a message relies on the recipient. Therefore a single evaluator introduces biases in meaning or numerical scoring, e.g. sentiment as a numbered scale. Additionally, the idea behind a group of people scoring text relies on Sir Francis Galton's theory of “Vox Populi” or wisdom of crowds.- Assessors need to exercise independent judgments.
- Assessors need to possess a diverse information understanding.
- Assessors need to rely on local knowledge.
- There has to be a way to tabulate the assessors' results.