
Graph Machine Learning
Claudio Stamile, Aldo Marzullo, Enrico Deusebio
- 338 Seiten
- English
- ePUB (handyfreundlich)
- Über iOS und Android verfügbar
Graph Machine Learning
Claudio Stamile, Aldo Marzullo, Enrico Deusebio
Über dieses Buch
Build machine learning algorithms using graph data and efficiently exploit topological information within your modelsKey Features• Implement machine learning techniques and algorithms in graph data• Identify the relationship between nodes in order to make better business decisions• Apply graph-based machine learning methods to solve real-life problemsBook DescriptionGraph Machine Learning will introduce you to a set of tools used for processing network data and leveraging the power of the relation between entities that can be used for predictive, modeling, and analytics tasks. The first chapters will introduce you to graph theory and graph machine learning, as well as the scope of their potential use. You'll then learn all you need to know about the main machine learning models for graph representation learning: their purpose, how they work, and how they can be implemented in a wide range of supervised and unsupervised learning applications. You'll build a complete machine learning pipeline, including data processing, model training, and prediction in order to exploit the full potential of graph data. After covering the basics, you'll be taken through real-world scenarios such as extracting data from social networks, text analytics, and natural language processing (NLP) using graphs and financial transaction systems on graphs. You'll also learn how to build and scale out data-driven applications for graph analytics to store, query, and process network information, and explore the latest trends on graphs. By the end of this machine learning book, you will have learned essential concepts of graph theory and all the algorithms and techniques used to build successful machine learning applications.What you will learn• Write Python scripts to extract features from graphs• Distinguish between the main graph representation learning techniques• Learn how to extract data from social networks, financial transaction systems, for text analysis, and more• Implement the main unsupervised and supervised graph embedding techniques• Get to grips with shallow embedding methods, graph neural networks, graph regularization methods, and more• Deploy and scale out your application seamlesslyWho this book is forThis book is for data scientists, data analysts, graph analysts, and graph professionals who want to leverage the information embedded in the connections and relations between data points to boost their analysis and model performance using machine learning. It will also be useful for machine learning developers or anyone who wants to build ML-driven graph databases. A beginner-level understanding of graph databases and graph data is required, alongside a solid understanding of ML basics. You'll also need intermediate-level Python programming knowledge to get started with this book.
Häufig gestellte Fragen
Information
Section 1 – Introduction to Graph Machine Learning
- Chapter 1, Getting Started with Graphs
- Chapter 2, Graph Machine Learning
Chapter 1: Getting Started with Graphs
- Introduction to graphs with networkx
- Plotting graphs
- Graph properties
- Benchmarks and repositories
- Dealing with large graphs
Technical requirements
- import networkx as nx
- import pandas as pd
- import numpy as np
Introduction to graphs with networkx










- The order of a graph is the number of its vertices |V|. The size of a graph is the number of its edges |E|.
- The degree of a vertex is the number of edges that are adjacent to it. The neighbors of a vertex v in a graph G is a subset of vertex
- The neighborhood graph (also known as an ego graph) of a vertex v in a graph G is a subgraph of G, composed of the vertices adjacent to v and all edges connecting vertices adjacent to v.
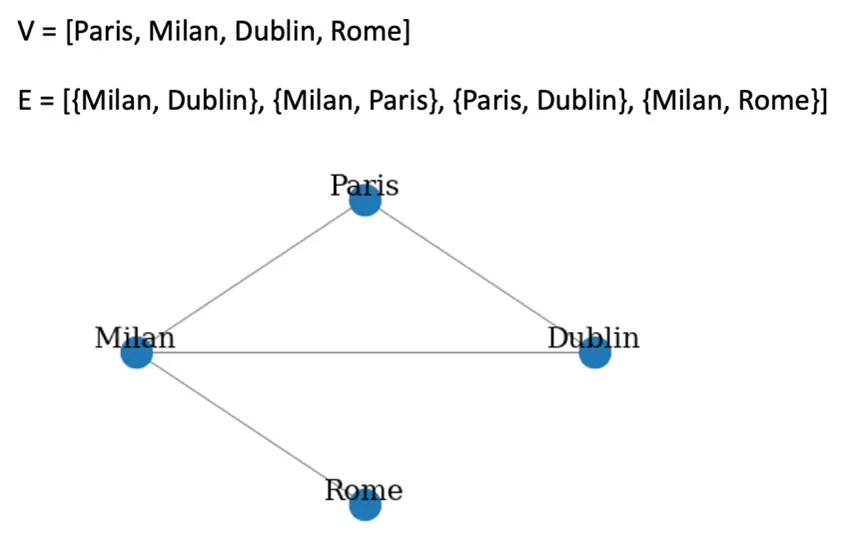