
Machine Learning with Amazon SageMaker Cookbook
Joshua Arvin Lat
- 762 Seiten
- English
- ePUB (handyfreundlich)
- Über iOS und Android verfügbar
Machine Learning with Amazon SageMaker Cookbook
Joshua Arvin Lat
Über dieses Buch
A step-by-step solution-based guide to preparing building, training, and deploying high-quality machine learning models with Amazon SageMakerKey Features• Perform ML experiments with built-in and custom algorithms in SageMaker• Explore proven solutions when working with TensorFlow, PyTorch, Hugging Face Transformers, and scikit-learn• Use the different features and capabilities of SageMaker to automate relevant ML processesBook DescriptionAmazon SageMaker is a fully managed machine learning (ML) service that helps data scientists and ML practitioners manage ML experiments. In this book, you'll use the different capabilities and features of Amazon SageMaker to solve relevant data science and ML problems. This step-by-step guide features 80 proven recipes designed to give you the hands-on machine learning experience needed to contribute to real-world experiments and projects. You'll cover the algorithms and techniques that are commonly used when training and deploying NLP, time series forecasting, and computer vision models to solve ML problems. You'll explore various solutions for working with deep learning libraries and frameworks such as TensorFlow, PyTorch, and Hugging Face Transformers in Amazon SageMaker. You'll also learn how to use SageMaker Clarify, SageMaker Model Monitor, SageMaker Debugger, and SageMaker Experiments to debug, manage, and monitor multiple ML experiments and deployments. Moreover, you'll have a better understanding of how SageMaker Feature Store, Autopilot, and Pipelines can meet the specific needs of data science teams. By the end of this book, you'll be able to combine the different solutions you've learned as building blocks to solve real-world ML problems.What you will learn• Train and deploy NLP, time series forecasting, and computer vision models to solve different business problems• Push the limits of customization in SageMaker using custom container images• Use AutoML capabilities with SageMaker Autopilot to create high-quality models• Work with effective data analysis and preparation techniques• Explore solutions for debugging and managing ML experiments and deployments• Deal with bias detection and ML explainability requirements using SageMaker Clarify• Automate intermediate and complex deployments and workflows using a variety of solutionsWho this book is forThis book is for developers, data scientists, and machine learning practitioners interested in using Amazon SageMaker to build, analyze, and deploy machine learning models with 80 step-by-step recipes. All you need is an AWS account to get things running. Prior knowledge of AWS, machine learning, and the Python programming language will help you to grasp the concepts covered in this book more effectively.
Häufig gestellte Fragen
Information
Chapter 1: Getting Started with Machine Learning Using Amazon SageMaker

- Launching an Amazon SageMaker notebook instance
- Checking the versions of the SageMaker Python SDK and the AWS CLI
- Preparing the Amazon S3 bucket and the training dataset for the linear regression experiment
- Visualizing and understanding your data in Python
- Training your first model in Python
- Loading a Linear Learner model with Apache MXNet in Python
- Evaluating the model in Python
- Deploying your first model in Python
- Invoking an Amazon SageMaker model endpoint with the SageMakerRuntime client from boto3
Technical requirements
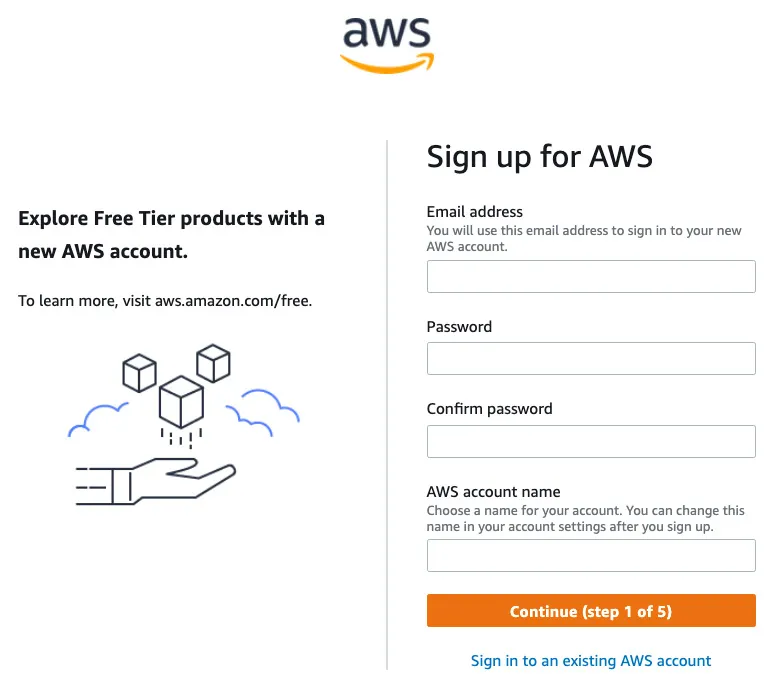