
Java Deep Learning Projects
Implement 10 real-world deep learning applications using Deeplearning4j and open source APIs
Md. Rezaul Karim
- 436 Seiten
- English
- ePUB (handyfreundlich)
- Über iOS und Android verfügbar
Java Deep Learning Projects
Implement 10 real-world deep learning applications using Deeplearning4j and open source APIs
Md. Rezaul Karim
Über dieses Buch
Build and deploy powerful neural network models using the latest Java deep learning libraries
Key Features
- Understand DL with Java by implementing real-world projects
- Master implementations of various ANN models and build your own DL systems
- Develop applications using NLP, image classification, RL, and GPU processing
Book Description
Java is one of the most widely used programming languages. With the rise of deep learning, it has become a popular choice of tool among data scientists and machine learning experts.
Java Deep Learning Projects starts with an overview of deep learning concepts and then delves into advanced projects. You will see how to build several projects using different deep neural network architectures such as multilayer perceptrons, Deep Belief Networks, CNN, LSTM, and Factorization Machines.
You will get acquainted with popular deep and machine learning libraries for Java such as Deeplearning4j, Spark ML, and RankSys and you'll be able to use their features to build and deploy projects on distributed computing environments.
You will then explore advanced domains such as transfer learning and deep reinforcement learning using the Java ecosystem, covering various real-world domains such as healthcare, NLP, image classification, and multimedia analytics with an easy-to-follow approach. Expert reviews and tips will follow every project to give you insights and hacks.
By the end of this book, you will have stepped up your expertise when it comes to deep learning in Java, taking it beyond theory and be able to build your own advanced deep learning systems.
What you will learn
- Master deep learning and neural network architectures
- Build real-life applications covering image classification, object detection, online trading, transfer learning, and multimedia analytics using DL4J and open-source APIs
- Train ML agents to learn from data using deep reinforcement learning
- Use factorization machines for advanced movie recommendations
- Train DL models on distributed GPUs for faster deep learning with Spark and DL4J
- Ease your learning experience through 69 FAQs
Who this book is for
If you are a data scientist, machine learning professional, or deep learning practitioner keen to expand your knowledge by delving into the practical aspects of deep learning with Java, then this book is what you need! Get ready to build advanced deep learning models to carry out complex numerical computations. Some basic understanding of machine learning concepts and a working knowledge of Java are required.
Häufig gestellte Fragen
Information
Developing Movie Recommendation Systems Using Factorization Machines
- Recommendation systems
- Matrix factorization and the collaborative filtering approach
- Developing FM-based move recommendation systems
- Frequently asked questions (FAQs).
Recommendation systems
Recommendation approaches
Collaborative filtering approaches
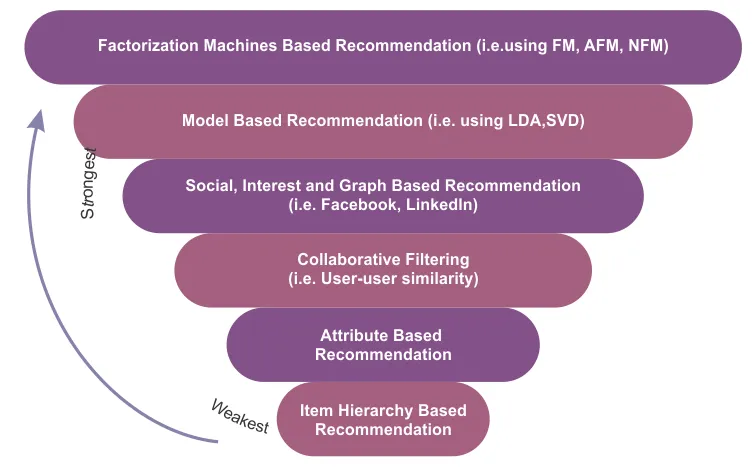
- Cold start: Sometimes, they can become stuck when a large amount of data about users is required to make a more accurate recommendation system.
- Scalability: A large amount of computation power is often necessary to calculate recommendations using a dataset with millions of users and products.
- Sparsity: This often happens with crowdsourced datasets when a huge number of items are sold on major e-commerce sites. All recommendation datasets are crowd-sourced in some sense. This is a general problem for almost all recommendation systems that have a sufficiently large number of items to offer to a sufficiently large number of users and need not be confined to e-commerce sites only.