
TensorFlow Machine Learning Cookbook
Over 60 recipes to build intelligent machine learning systems with the power of Python, 2nd Edition
Nick McClure
- 422 Seiten
- English
- ePUB (handyfreundlich)
- Über iOS und Android verfügbar
TensorFlow Machine Learning Cookbook
Over 60 recipes to build intelligent machine learning systems with the power of Python, 2nd Edition
Nick McClure
Über dieses Buch
Skip the theory and get the most out of Tensorflow to build production-ready machine learning models
Key Features
- Exploit the features of Tensorflow to build and deploy machine learning models
- Train neural networks to tackle real-world problems in Computer Vision and NLP
- Handy techniques to write production-ready code for your Tensorflow models
Book Description
TensorFlow is an open source software library for Machine Intelligence. The independent recipes in this book will teach you how to use TensorFlow for complex data computations and allow you to dig deeper and gain more insights into your data than ever before.
With the help of this book, you will work with recipes for training models, model evaluation, sentiment analysis, regression analysis, clustering analysis, artificial neural networks, and more. You will explore RNNs, CNNs, GANs, reinforcement learning, and capsule networks, each using Google's machine learning library, TensorFlow. Through real-world examples, you will get hands-on experience with linear regression techniques with TensorFlow. Once you are familiar and comfortable with the TensorFlow ecosystem, you will be shown how to take it to production.
By the end of the book, you will be proficient in the field of machine intelligence using TensorFlow. You will also have good insight into deep learning and be capable of implementing machine learning algorithms in real-world scenarios.
What you will learn
- Become familiar with the basic features of the TensorFlow library
- Get to know Linear Regression techniques with TensorFlow
- Learn SVMs with hands-on recipes
- Implement neural networks to improve predictive modeling
- Apply NLP and sentiment analysis to your data
- Master CNN and RNN through practical recipes
- Implement the gradient boosted random forest to predict housing prices
- Take TensorFlow into production
Who this book is for
If you are a data scientist or a machine learning engineer with some knowledge of linear algebra, statistics, and machine learning, this book is for you. If you want to skip the theory and build production-ready machine learning models using Tensorflow without reading pages and pages of material, this book is for you. Some background in Python programming is assumed.
Häufig gestellte Fragen
Information
Recurrent Neural Networks
- Implementing RNNs for spam prediction
- Implementing an LSTM model
- Stacking multiple LSTM layers
- Creating sequence-to-sequence models
- Training a Siamese similarity measure
Introduction
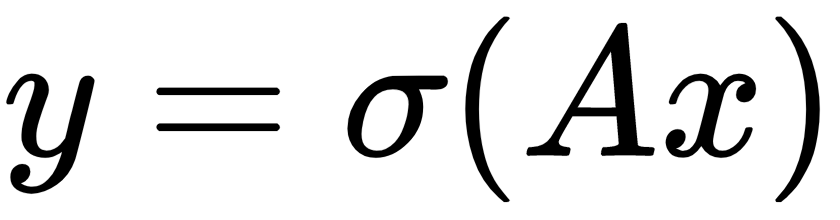





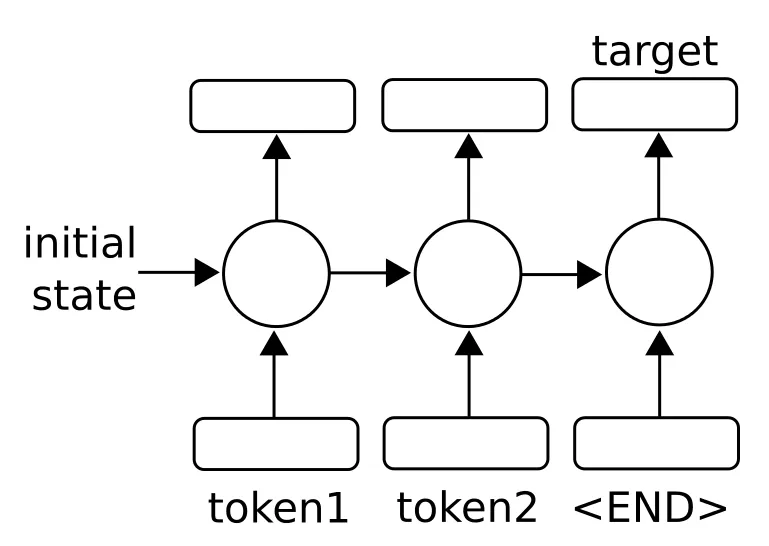

Implementing RNN for spam prediction
Getting ready
How to do it...
- We start by loading the libraries required for this script:
import os import re import io import requests import numpy as np import matplotlib.pyplot as plt import tensorflow as tf from zipfile import ZipFile
- Next, we start a graph session and set the RNN model parameters. We will run the data through 20 epochs, in batch sizes of 250. The maximum length of each text we will consider is 25 words; we will cut longer texts to 25 or zero-pad shorter texts. The RNN will be size 10 units. We will only consider words that appear at least 10 times in our vocabulary, and every word will be embedded in a trainable vector of size 50. The dropout rate will be a placeholder that we can set at 0.5 during training time or 1.0 during evaluation:
sess = tf.Session() epochs = 20 batch_size = 250 max_sequence_length = 25 rnn_size = 10 embedding_size = 50 min_word_frequency = 10 learning_rate = 0.0005 dropout_keep_prob = tf.placeholder(tf.float32)
- Now we get the SMS text data. First, we check whether it has already been downloaded and, if so, read in the file. Otherwise, we download the data and save it:
data_dir = 'temp' data_file = 'text_data.txt' if not os.path.exists(data_dir): os.makedirs(data_dir) if not os.path.isfile(os.path.join(data_dir, data_file)): zip_url = 'http://archive.ics.uci.edu/ml/machine-learning-databases/00228/smsspamcollection.zip' r = requests.get(zip_url) z = ZipFile(io.BytesIO(r.content)) file = z.read('SMSSpamCollection') # Format Data text_data = file.decode() text_data = text_data.encode('ascii',errors='ignore') text_data = text_data.decode().split('\n') # Save data to text file with open(os.path.join(data_dir, data_file), 'w') as file_conn: for text in text_data: file_conn.write("{}\n".format(text)) else: # Open data from text file text_data = [] with open(os.path.join(data_dir, data_file), 'r') as file_conn: for row in file_conn: text_data.append(row) text_data = text_data[:-1] text_data = [x.split('\t') for x in text_data if len(x)>=1] [text_data_target, text_data_train] = [list(x) for x in zip(*text_data)]
- To reduce our vocabulary, we will clean the input text by removing special characters, and extra white space, and putting everything in lowercase:
def clean_text(text_string):
text_string = re.sub(r'([^sw]|_|[0-9])+', '', text_string)
text_string = " ".join(text_string.split())
text_string = text_string.lower()
return text_string
# Clean texts
text_data_train = [clean_text(x) for x in text_data_train]
- Now we process the text with a built-in vocabulary processor function from TensorFlow. This will convert the text to an appropriate list of indices:
vocab_processor = tf.contrib.learn.preprocessing.VocabularyProcessor(max_sequence_length, min_frequency=min_word_frequency) text_processed = np.array(list(vocab_processor.fit_transform(text_data_train)))
- Next, we shuffle the data to randomize it:
text_processed = np.array(text_processed) text_data_target = np.array([1 if x=='ham' else 0 for x in text_data_target]) shuffled_ix = np.random.permutation(np.arange(len(text_data_target))) x_shuffled = text_processed[shuffled_ix] y_shuffled = text_data_target[shuffled_ix]
- We also split the data into an 80-20 train-test dataset:
ix_cutoff = int(len(y_shuffled)*0.80) x_train, x_test = x_shuffled[:ix_cutoff], x_shuffled[ix_cutoff:] y_train, y_test = y_shuffled[:ix_cutoff], y_shuffled[ix_cutoff:] vocab_size = len(vocab_processor.vocabulary_) print("Vocabulary Size: {:d}".format(vocab_size)) print("80-20 Train Test split: {:d} -- {:d}".format(len(y_train), len(y_test)))