
The Digital Agricultural Revolution
Innovations and Challenges in Agriculture through Technology Disruptions
Roheet Bhatnagar, Nitin Kumar Tripathi, Nitu Bhatnagar, Chandan Kumar Panda, Roheet Bhatnagar, Nitin Kumar Tripathi, Nitu Bhatnagar, Chandan Kumar Panda
- English
- ePUB (adapté aux mobiles)
- Disponible sur iOS et Android
The Digital Agricultural Revolution
Innovations and Challenges in Agriculture through Technology Disruptions
Roheet Bhatnagar, Nitin Kumar Tripathi, Nitu Bhatnagar, Chandan Kumar Panda, Roheet Bhatnagar, Nitin Kumar Tripathi, Nitu Bhatnagar, Chandan Kumar Panda
Ă propos de ce livre
THE DIGITAL AGRICULTURAL REVOLUTION
The book integrates computational intelligence, applied artificial intelligence, and modern agricultural practices and will appeal to scientists, agriculturists, and those in plant and crop science management.
There is a need for synergy between the application of modern scientific innovation in the area of artificial intelligence and agriculture, considering the major challenges from climate change consequences viz. rising temperatures, erratic rainfall patterns, the emergence of new crop pests, drought, flood, etc. This volume reports on high-quality research (theory and practice including prototype & conceptualization of ideas, frameworks, real-world applications, policy, standards, psychological concerns, case studies, and critical surveys) on recent advances toward the realization of the digital agriculture revolution as a result of the convergence of different disruptive technologies.
The book touches upon the following topics which have contributed to revolutionizing agricultural practices.
- Applications of Artificial Intelligence in Agriculture (AI models and architectures, system design, real-world applications of AI, machine learning and deep learning in the agriculture domain, integration & coordination of systems and issues & challenges).
- IoT and Big Data Analytics Applications in Agriculture (theory & architecture and the use of various types of sensors in optimizing agriculture resources and final product, benefits in real-time for crop acreage estimation, monitoring & control of agricultural produce).
- Robotics & Automation in Agriculture Systems (Automation challenges, need and recent developments and real case studies).
- Intelligent and Innovative Smart Agriculture Applications (use of hybrid intelligence in better crop health and management).
- Privacy, Security, and Trust in Digital Agriculture (government framework & policy papers).
- Open Problems, Challenges, and Future Trends.
Audience
Researchers in computer science, artificial intelligence, electronics engineering, agriculture automation, crop management, and science.
Foire aux questions
Informations
1
Scope and Recent Trends of Artificial Intelligence in Indian Agriculture
AbstractAgriculture is the economic backbone of India. About 6.4% of the total worldâs economy relies on agriculture [1]. Automation in agriculture is the emerging sector as there is an increase in food demand and employment. The traditional ways used by farmers are not sufficient to fulfill the demands and it is high time that newer technologies are implementing in the agricultural sector. Artificial Intelligence (AI) is one of the emerging and promising technologies where intelligence refers to developing and utilizing human-level thinking machines through learning algorithms programmed to solve critical problems. Artificial Intelligence plays an important role in supporting agriculture sectors with the objectives of boosting productivity, efficiency, and farmersâ income. This chapter focuses on how AI helps in increasing the socioeconomic and environmental sustainability in the Indian agricultural sector. Also, it highlights the AI practices in India incorporated by farmers having small and medium-size agricultural lands.Keywords: Indian agriculture, Artificial Intelligence, farmers
1.1 Introduction
1.2 Different Forms of AI
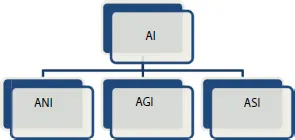
1.3 Different Technologies in AI
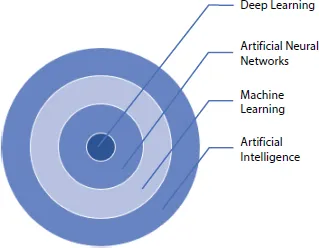
AI | AI is a technology that allows us to build intelligent systems that mimic human intelligence. |
ML | ML is an AI discipline that allows machines to learn from previous data or experiences without having to be explicitly programmed. |
ANN | ANN depends on algorithms resembling the human brain. |
DL | DL algorithms automatically build a hierarchy of data representations using the low- and high-level features. |
1.3.1 Machine Learning
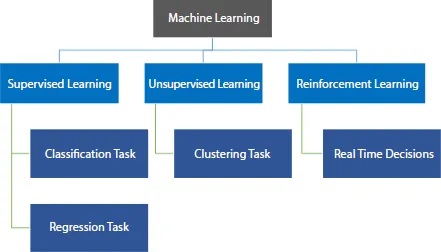
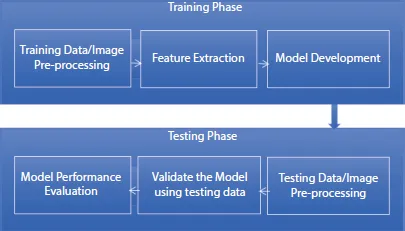