
Big Data Analytics with Hadoop 3
Build highly effective analytics solutions to gain valuable insight into your big data
Sridhar Alla
- 482 pagine
- English
- ePUB (disponibile sull'app)
- Disponibile su iOS e Android
Big Data Analytics with Hadoop 3
Build highly effective analytics solutions to gain valuable insight into your big data
Sridhar Alla
Informazioni sul libro
Explore big data concepts, platforms, analytics, and their applications using the power of Hadoop 3About This Book• Learn Hadoop 3 to build effective big data analytics solutions on-premise and on cloud• Integrate Hadoop with other big data tools such as R, Python, Apache Spark, and Apache Flink• Exploit big data using Hadoop 3 with real-world examplesWho This Book Is ForBig Data Analytics with Hadoop 3 is for you if you are looking to build high-performance analytics solutions for your enterprise or business using Hadoop 3's powerful features, or you're new to big data analytics. A basic understanding of the Java programming language is required.What You Will Learn• Explore the new features of Hadoop 3 along with HDFS, YARN, and MapReduce• Get well-versed with the analytical capabilities of Hadoop ecosystem using practical examples• Integrate Hadoop with R and Python for more efficient big data processing• Learn to use Hadoop with Apache Spark and Apache Flink for real-time data analytics• Set up a Hadoop cluster on AWS cloud• Perform big data analytics on AWS using Elastic Map ReduceIn DetailApache Hadoop is the most popular platform for big data processing, and can be combined with a host of other big data tools to build powerful analytics solutions. Big Data Analytics with Hadoop 3 shows you how to do just that, by providing insights into the software as well as its benefits with the help of practical examples.Once you have taken a tour of Hadoop 3's latest features, you will get an overview of HDFS, MapReduce, and YARN, and how they enable faster, more efficient big data processing. You will then move on to learning how to integrate Hadoop with the open source tools, such as Python and R, to analyze and visualize data and perform statistical computing on big data. As you get acquainted with all this, you will explore how to use Hadoop 3 with Apache Spark and Apache Flink for real-time data analytics and stream processing. In addition to this, you will understand how to use Hadoop to build analytics solutions on the cloud and an end-to-end pipeline to perform big data analysis using practical use cases.By the end of this book, you will be well-versed with the analytical capabilities of the Hadoop ecosystem. You will be able to build powerful solutions to perform big data analytics and get insight effortlessly.Style and approachFilled with practical examples and use cases, this book will not only help you get up and running with Hadoop, but will also take you farther down the road to deal with Big Data Analytics
Domande frequenti
Informazioni
Big Data Processing with MapReduce
- The MapReduce framework
- MapReduce job types:
- Single mapper jobs
- Single mapper reducer jobs
- Multiple mappers reducer jobs
- MapReduce patterns:
- Aggregation patterns
- Filtering patterns
- Join patterns
The MapReduce framework

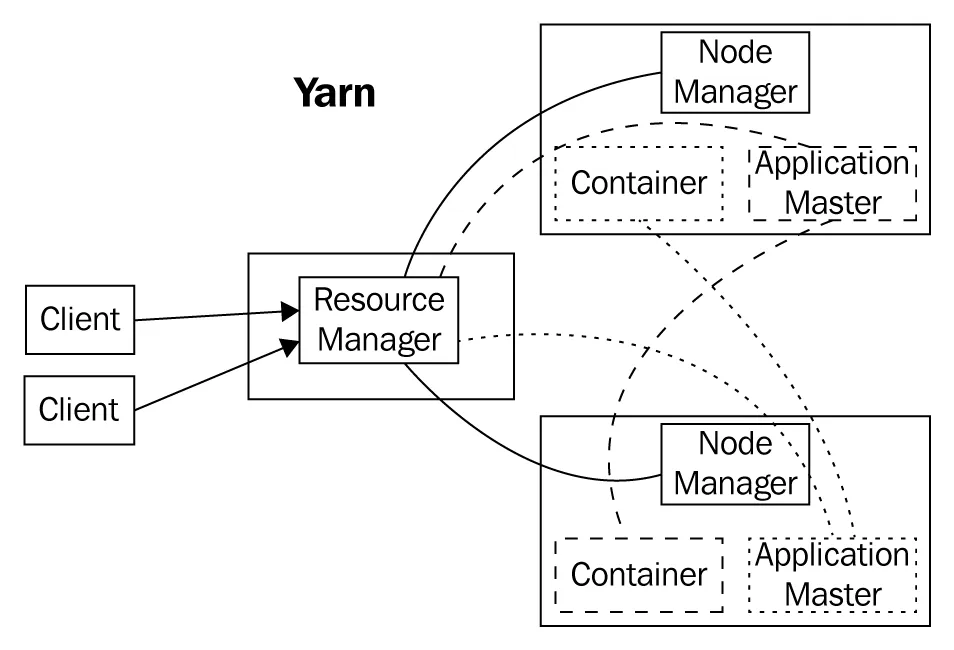
Dataset
Id,City
1,Boston
2,New York
3,Chicago
4,Philadelphia
5,San Francisco
7,Las Vegas
hdfs dfs -copyFromLocal cities.csv /user/normal
Date,Id,Temperature
2018-01-01,1,21
2018-01-01,2,22
2018-01-01,3,23
2018-01-01,4,24
2018-01-01,5,25
2018-01-01,6,22
2018-01-02,1,23
2018-01-02,2,24
2018-01-02,3,25