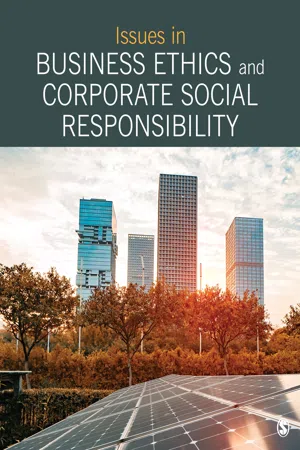
eBook - ePub
Issues in Business Ethics and Corporate Social Responsibility
Selections from SAGE Business Researcher
SAGE Publishing
This is a test
- 424 pages
- English
- ePUB (mobile friendly)
- Available on iOS & Android
eBook - ePub
Issues in Business Ethics and Corporate Social Responsibility
Selections from SAGE Business Researcher
SAGE Publishing
Book details
Book preview
Table of contents
Citations
About This Book
Issues in Business Ethics and Corporate Social Responsibility explores these foundational themes across a wide range of topics, including artificial intelligence, workplace surveillance, supply chain management, big data, the finance industry, and many more. Coupled with a broad introduction by Dr. David Weitzner, a professor of management at York University, this book provides students with the essential information they need to assess business practices through the lens of ethical decision-making and corporate social responsibility.
Frequently asked questions
How do I cancel my subscription?
Can/how do I download books?
At the moment all of our mobile-responsive ePub books are available to download via the app. Most of our PDFs are also available to download and we're working on making the final remaining ones downloadable now. Learn more here.
What is the difference between the pricing plans?
Both plans give you full access to the library and all of Perlego’s features. The only differences are the price and subscription period: With the annual plan you’ll save around 30% compared to 12 months on the monthly plan.
What is Perlego?
We are an online textbook subscription service, where you can get access to an entire online library for less than the price of a single book per month. With over 1 million books across 1000+ topics, we’ve got you covered! Learn more here.
Do you support text-to-speech?
Look out for the read-aloud symbol on your next book to see if you can listen to it. The read-aloud tool reads text aloud for you, highlighting the text as it is being read. You can pause it, speed it up and slow it down. Learn more here.
Is Issues in Business Ethics and Corporate Social Responsibility an online PDF/ePUB?
Yes, you can access Issues in Business Ethics and Corporate Social Responsibility by SAGE Publishing in PDF and/or ePUB format, as well as other popular books in Business & Etica aziendale. We have over one million books available in our catalogue for you to explore.
Information
1 AI and Ethics: Can Machines Learn to Explain their Decisions?
From SAGE Business Researcher, June 11, 2018
Executive Summary
Artificial intelligence, or AI—machine functions that exhibit abilities of the human mind such as learning and problem solving—is in a phase of rapid development. U.S.-based and international technology companies are in an intense competition to develop AI systems that can automate tasks in a diverse range of industries, from entertainment to marketing to human resources. However, AI systems are so complex that even their developers are sometimes uncertain about how they make decisions. This has raised unique ethical and regulatory challenges, as researchers point to evidence that AI systems can be biased in favor of certain groups of people for jobs, services or loans. Many researchers are calling for greater transparency and for a legal “right to explanation” for those affected by AI decisions.
Here are some key takeaways:
- Investments in AI are being fueled by tech giants, such as Google and Baidu; companies invested more than $20 billion in AI-related mergers and acquisitions globally last year.
- There has also been an upswing in research into AI systems such as deep learning, with most of it published in China and the United States.
- AI’s decision-making in high-stakes fields such as hiring and criminal justice has elevated ethical concerns about bias and accountability.
Overview
Henry Gan, a software engineer at the short-video hosting company Gfycat, tested new software on his coworkers last year that used artificial intelligence to identify their faces. While the software correctly matched most of Gan’s coworkers with their names, it confused Asian faces.1
Gan, who is Asian-American, attempted to remedy the issue by adding more photos of Asians and darker-skinned celebrities into the database that “trained” the software. His efforts produced little improvement. So Gan and his colleagues built a feature that forced the software to apply a more rigorous standard for determining a match when it recognized a face sharing features similar to Asians in its database. Gan acknowledged that allowing the software to look for racial differences to counteract prejudice may seem counterintuitive, but he said it “was the only way to get it to not mark every Asian person as Jackie Chan or something.”2
Artificial intelligence, or AI, is in a phase of rapid development. Tech giants such as Google and Baidu are pouring money into it; in 2017, companies globally invested around $21.3 billion in AI-related mergers and acquisitions, according to PitchBook, a private market data provider.3 Yet amid this investment growth, AI systems have presented unique ethical and regulatory challenges as their real-life applications, such as in facial recognition software, have shown evidence of bias.
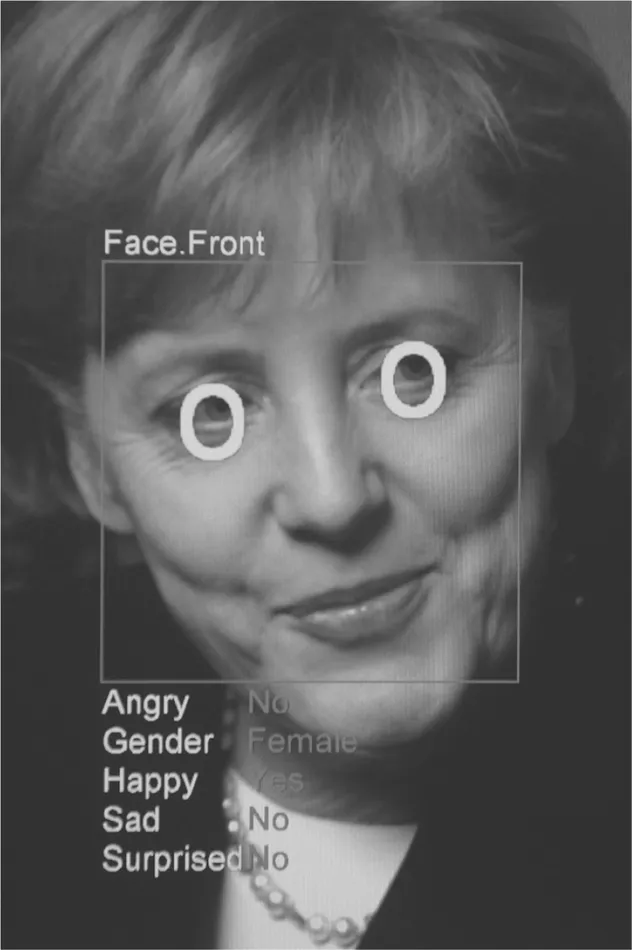
AI powers facial recognition software, such as this system that analyzed a photo of German Chancellor Angela Merkel.
John MacDougall/AFP/Getty Images
AI research was born as an academic discipline from a workshop at Dartmouth College in 1956.4 After several cycles of high expectations followed by disappointment and loss of research funding, the current wave of progress accelerated in 2010 with the availability of big data, increased computer processing power and advancements in machine learning algorithms.5
“The most important general-purpose technology of our era is artificial intelligence, particularly machine learning … that is, the machine’s ability to keep improving its performance without humans having to explain exactly how to accomplish all the tasks it’s given,” wrote Erik Brynjolfsson and Andrew McAfee, co-directors of the Massachusetts Institute of Technology’s Initiative on the Digital Economy.6
Machine learning differs from the previous approach to AI, in which developers had to explicitly code rules into software. Instead, machine learning allows a system to learn from examples, find patterns, make predictions and improve its own performance. Deep learning, which is a subfield of machine learning, enables a system to process information in layers to accurately recognize extremely complex data patterns. Researchers have been surprised by the recent successes of very large deep-learning networks, which is the main cause of current optimism for AI.7
In 2016, machine learning attracted $5 billion to $7 billion in investment, according to a report by the global management company McKinsey.8 Machine learning can be combined with other technologies to enable a wide range of tasks. The entertainment company Netflix reported how it used machine learning algorithms to personalize video-streaming options for its subscribers and avoid cancelled subscriptions that saved the company $1 billion per year.9 The marketing company Infinite Analytics used machine learning to improve online advertising placement for its clients, which resulted in a threefold return on investment for a global packaged goods company.10
Companies are also integrating machine learning into their business processes, including chatbots that automate customer service or smart robots that are designed to collaborate with human workers in factories and warehouses.11 In 2012, the e-commerce giant Amazon acquired the robotics company Kiva, and used machine learning to improve the performance of Amazon’s 80,000 robots in its fulfillment centers to reduce operating costs by 20 percent.12
“The methodologies and hardware to support machine learning have become unbelievably advanced,” says Michael Skirpan, co-founder of the self-described ethical engineering consulting and research firm Probable Models, who provides training to companies’ engineering teams about fairness in machine learning. “Most adopters are large-enterprise companies that are putting machine learning into their software or online platforms.”

Companies Adopt AI to Stay Competitive
Source: Louis Columbus, “How Artificial Intelligence Is Revolutionizing Business In 2017,” Forbes, Sept. 10, 2017, https://tinyurl.com/ybvo3fsj
Most investment in AI has consisted of internal spending by large U.S.-based and international technology companies, which are engaged in intense competition to lead AI innovation. One measure of this interest is the sharp increase from 2013 to 2015 in journal articles mentioning “deep learning,” with most of the research published in the United States and China. Another measure is that deep-learning patents increased sixfold in that time.13 The excitement of AI comes from its immense potential to systemically transform industries, according to Brynjolfsson and McAfee.14
Currently, however, machine learning is still considered to be early in its development as a research field. Technology companies are staking out their positions in this phase of AI deployment and securing the limited pool of AI talent by acquiring startups and recruiting top researchers from universities by offering them higher salaries, company stock options and the opportunity to work with large, privately held datasets.15
As competition in AI intensifies, academic institutions and researchers as well as various organizations are focusing more attention on the technology’s ethical and social effects. While the performance of AI systems has improved rapidly in the closed world of the laboratory, the transition of AI into real-world applications has raised unforeseen challenges, such as how AI systems can make decisions that reflect and reinforce human prejudices.16
Fairness in AI and Machine Learning
An AI system can learn bias in a number of ways, according to researchers in a Microsoft team called FATE (for fairness, accountability, transparency and ethics). The system can mimic behaviors it learns from its users, it can be trained with incomplete or historically prejudiced datasets, or its code can reflect the biases of the developers who had built them, the researchers concluded.17
Hiring is a prime example of how an AI system can learn bias from the quality of the data on which it is trained. In this area, the system can automate the initial assessment of job applicants and make retention predictions to reduce turnover costs for a company. Solon Barocas, an assistant professor of information science at Cornell University, explains that the AI system would first have to be trained using historical data. By exposing the system to examples of past job applicants who have gone on to be high- or low-performing employees, the system will attempt to learn the distinguishing characteristics of each, in order to make performance predictions about future job applicants. “The hope is that computers can be much more powerful in finding those indicators of potentially high-performing employees,” Barocas says.
However, because the data used to train the system come from managers’ evaluations, “what the machine learning model is learning to predict is not who is going to perform well at the job, but … managers’ evaluations of these people,” Barocas says. If the managers’ assessments were biased, the AI system will learn discriminatory practices inherent in a historic dataset and apply those to future candidates.
Bias can also occur when an AI system is trained on data that are not representative of the people who will be affected by the algorithms. In hiring, if an employer had categorically discriminated against certain groups of people for positions, the system will continue to reject those applicants, simply because it has no data about them. Will Byrne, director of strategy at Presence Product Group, a product studio specializing in digital technology, wrote, “As a result of societal bias and lack of equal opportunity, predictors of successful women engineers and predictors of successful male engineers are simply not the same. Creating different algorithms with unique training corpuses for different groups … leaves a minority group less disadvantaged.”18
Applications of AI in Commercial and Public Institutions
Algorithmic bias draws further concern from researchers as an increasing number of social, corporate and governmental institutions implement AI systems to automate decisions.1...
Table of contents
- Cover
- Half Title
- Publisher Note
- Title Page
- Copyright Page
- Brief Contents
- Detailed Contents
- About the Authors
- Introduction
- 1 AI and Ethics: Can Machines Learn to Explain their Decisions?
- 2 Business and Climate Change: Can the International Business Community Adapt to the Risks?
- 3 Business and Universities: Should Academic Capitalism Shape Teaching and Research?
- 4 China’s Big Data Sweep: Will Beijing Push the Boundaries of Social Control?
- 5 Corporate Social Responsibility: Are top-profile issues good for the bottom line?
- 6 Ethics and Financial Services: Has the industry learned from the global crisis?
- 7 Ethics and the Supply Chain: Can businesses police the behavior of global suppliers?
- 8 Managing Corporate Crises: Can companies contain damage in the social-media age?
- 9 Sexual Harassment: Will employers act effectively to curb it?
- 10 Sustainability: Are businesses looking beyond profit?
- 11 Tax Havens: Can regulators catch up with offshore shelters?
- 12 Technology and Business Ethics: Can companies resist wrongdoing in a digital world?
- 13 The Boycott: Can it bring the powerful to heel?
- 14 The Fiduciary Rule: Should advisers be required to put clients first?
- 15 The Gun Industry: Will corporate action force change on gunmakers?
- 16 Workplace Safety: Is Trump gutting on-the-job protections?
- 17 Workplace Surveillance: Will new techniques spark resentment?
- References