
Machine Learning Algorithms
Popular algorithms for data science and machine learning, 2nd Edition
Giuseppe Bonaccorso
- 522 Seiten
- English
- ePUB (handyfreundlich)
- Über iOS und Android verfügbar
Machine Learning Algorithms
Popular algorithms for data science and machine learning, 2nd Edition
Giuseppe Bonaccorso
Über dieses Buch
An easy-to-follow, step-by-step guide for getting to grips with the real-world application of machine learning algorithms
Key Features
- Explore statistics and complex mathematics for data-intensive applications
- Discover new developments in EM algorithm, PCA, and bayesian regression
- Study patterns and make predictions across various datasets
Book Description
Machine learning has gained tremendous popularity for its powerful and fast predictions with large datasets. However, the true forces behind its powerful output are the complex algorithms involving substantial statistical analysis that churn large datasets and generate substantial insight.
This second edition of Machine Learning Algorithms walks you through prominent development outcomes that have taken place relating to machine learning algorithms, which constitute major contributions to the machine learning process and help you to strengthen and master statistical interpretation across the areas of supervised, semi-supervised, and reinforcement learning. Once the core concepts of an algorithm have been covered, you'll explore real-world examples based on the most diffused libraries, such as scikit-learn, NLTK, TensorFlow, and Keras. You will discover new topics such as principal component analysis (PCA), independent component analysis (ICA), Bayesian regression, discriminant analysis, advanced clustering, and gaussian mixture.
By the end of this book, you will have studied machine learning algorithms and be able to put them into production to make your machine learning applications more innovative.
What you will learn
- Study feature selection and the feature engineering process
- Assess performance and error trade-offs for linear regression
- Build a data model and understand how it works by using different types of algorithm
- Learn to tune the parameters of Support Vector Machines (SVM)
- Explore the concept of natural language processing (NLP) and recommendation systems
- Create a machine learning architecture from scratch
Who this book is for
Machine Learning Algorithms is for you if you are a machine learning engineer, data engineer, or junior data scientist who wants to advance in the field of predictive analytics and machine learning. Familiarity with R and Python will be an added advantage for getting the best from this book.
Häufig gestellte Fragen
Information
Linear Classification Algorithms
- The general structure of a linear classification problem
- Logistic regression (with and without regularization)
- SGD algorithms and perceptron
- Passive-aggressive algorithms
- Grid search of optimal hyperparameters
- The most important classification metrics
- The Receiver Operating Characteristic (ROC) curve
Linear classification








Logistic regression

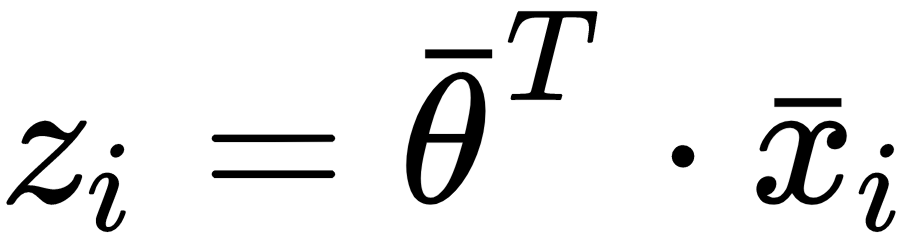
